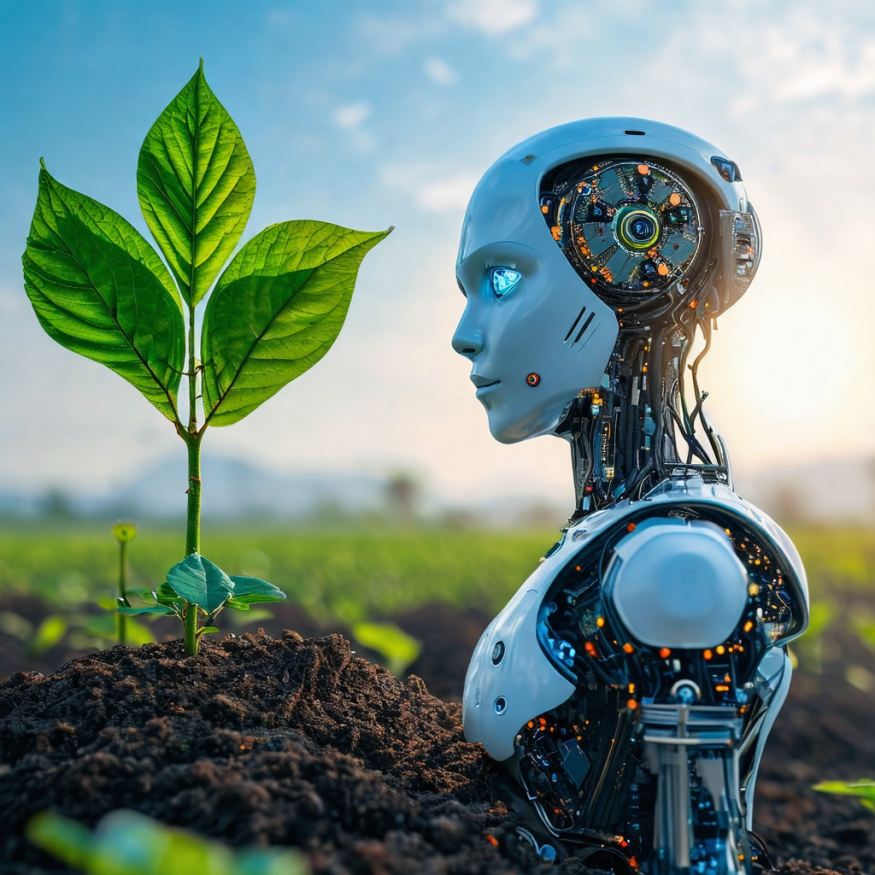
Abstract: Generative AI for a Sustainable Future examines the transformative potential of generative artificial intelligence (AI) in advancing sustainability across diverse sectors. By leveraging generative AI's unique capabilities in simulation, optimization, and creation, industries can make strides toward achieving Sustainable Development Goals (SDGs) through enhanced energy efficiency, resource management, and climate change mitigation. The paper explores the role of generative AI in reducing carbon emissions, optimizing renewable energy systems, and supporting conservation efforts, all while addressing the technology's inherent challenges, such as high computational demands, data privacy, and ethical considerations. Through practical applications and case studies, this article demonstrates how generative AI can support environmental, social, and governance (ESG) initiatives, highlighting the need for responsible AI deployment strategies to maximize sustainable impact. Looking forward, generative AI's integration into sectors like drug design, architecture, and engineering showcases its potential as a catalyst for sustainable development and innovation.
Keywords: Generative AI, Sustainability, Sustainable Development Goals (SDGs), Energy efficiency, Resource management, Climate change mitigation, Environmental, Social, and Governance (ESG), Carbon emissions reduction, Ethical AI, Responsible AI, Machine learning in sustainability, Conservation technology, Renewable energy optimization, AI for biodiversity, Data privacy in AI
Generative AI for a sustainable future explores the transformative role of generative artificial intelligence in advancing sustainability across various sectors. As a branch of machine learning, generative AI has the unique capability to create, simulate, and optimize complex systems, offering innovative solutions that can significantly contribute to environmental conservation and the achievement of Sustainable Development Goals (SDGs)[1]. By reducing resource consumption and enhancing efficiency, this technology presents a promising pathway to mitigate some of the most pressing environmental challenges[1].
Generative AI is notably impactful in areas such as energy efficiency, renewable energy, and resource management. It aids in optimizing energy consumption, thereby reducing carbon emissions and facilitating the transition to cleaner energy sources[2]. In resource management, AI applications enable precise monitoring and conservation efforts in biodiversity and forestry, contributing to ecological balance[3]. Additionally, generative AI assists in climate change mitigation by improving weather prediction models and optimizing waste management systems, ultimately reducing global greenhouse gas emissions[4].
However, the deployment of generative AI is not without challenges. The technology's substantial computing requirements raise concerns about its energy consumption and carbon footprint, which necessitate strategies to minimize environmental impact while maximizing sustainability benefits[5]. Moreover, data privacy, ethical considerations, and potential biases in AI systems are significant issues that require careful governance and oversight to ensure that the implementation of generative AI aligns with ethical standards and sustainability goals[6].
The future of generative AI in sustainability is promising, with potential applications extending into fields like drug design, architecture, and engineering. These advancements are expected to bolster efforts in environmental, social, and governance (ESG) initiatives, reinforcing the value of AI as a tool for sustainable development[7]. As industries increasingly integrate sustainability into their operational frameworks, generative AI stands out as a pivotal technology that can drive innovation while upholding environmental integrity[8].
Applications of Generative AI in Sustainability
Generative AI has emerged as a powerful tool for promoting sustainability across various sectors, offering innovative solutions that can help protect the planet while advancing the Sustainable Development Goals (SDGs)[1]. The technology's ability to create, simulate, and optimize has untapped potential in fostering sustainable practices.
Energy Efficiency and Renewable Energy
One of the significant applications of generative AI in sustainability is in the field of energy efficiency and renewable energy. By optimizing energy consumption and enhancing the efficiency of renewable energy systems, generative AI contributes to the reduction of carbon emissions and supports the transition to cleaner energy sources[1]. Enterprises are encouraged to partner with vendors that prioritize reducing power consumption and leverage high-quality renewable energy, aligning with sustainability goals[2].
Resource Management and Conservation
Generative AI plays a crucial role in sustainable resource management by helping to scale AI solutions for managing resources efficiently[1]. In the forestry and biodiversity sectors, AI applications are used for effective monitoring, management, and conservation efforts. These technologies enable precise tracking of biodiversity and forest resources, enhancing the efforts to maintain ecological balance[3].
Climate Change Mitigation
AI's potential in combating climate change is evident through applications in various environmental systems. AI tools are instrumental in predicting weather patterns, tracking icebergs, and identifying pollution, which aids in mitigating the adverse impacts of climate change[4]. Moreover, generative AI is applied in waste management systems to improve efficiency, thereby reducing methane emissions and contributing to the reduction of global greenhouse gas emissions[4].
Challenges and Considerations
Generative AI offers transformative potential for a sustainable future, yet it presents several challenges and considerations that need careful attention. One of the primary concerns revolves around data privacy and security. The vast amounts of data consumed by generative AI systems can be inadequately governed, originating from sources that are potentially biased or used without consent, which can lead to biased outputs and privacy issues[9][10]. Furthermore, user data might be sold or given to third parties for marketing or surveillance, necessitating caution in sharing sensitive information with AI tools[5].
The environmental impact of AI technologies is another critical consideration. While generative AI can aid sustainable development, it also contributes to environmental challenges through its substantial computational power requirements, leading to increased carbon emissions and electronic waste[8]. Balancing the benefits of AI against its environmental footprint is crucial for its sustainable deployment.
From an ethical standpoint, generative AI poses significant risks. The automatic generation of content can perpetuate biases inherent in training data, resulting in harmful or biased language that requires human oversight to align with ethical business practices[10]. Moreover, the adoption of generative AI systems affects organizational structures and workforce dynamics, necessitating strategic planning to mitigate negative impacts while leveraging potential growth[9].
To navigate these challenges, it is essential to prioritize AI trust and transparency technologies. These solutions can enhance the governance of AI systems, ensuring ethical use and minimizing potential risks[2]. Additionally, conducting thorough assessments of AI models during the early stages of development can align their implementation with sustainability goals and ethical considerations[7][11].
Benefits of Generative AI for a Sustainable Future
Generative AI holds significant promise in advancing sustainable development across various sectors by leveraging its capabilities to create, simulate, and optimize complex systems. This transformative technology can address environmental challenges through innovative solutions, contributing to a more sustainable future[1]. By reducing manual efforts and enhancing efficiency, generative AI can significantly impact the management of sustainable resources, facilitating scalable solutions for environmental integrity[8].
One of the primary benefits of generative AI is its ability to improve agricultural practices by analyzing data from sensors placed on crops. This enables the reduction of environmental impact while boosting productivity[4]. Furthermore, AI technologies, including generative models, are already being utilized in initiatives like The Ocean Cleanup, where they play a crucial role in identifying and mitigating plastic pollution in marine environments[4].
However, it is important to acknowledge the environmental costs associated with generative AI. The technology demands substantial computing resources, which in turn contribute to carbon emissions. Therefore, balancing the technological benefits with these environmental impacts is essential[7]. Ongoing research and development are needed to optimize AI deployments to minimize their carbon footprint while maximizing their contribution to sustainability[7].
The potential applications of generative AI are vast, with future prospects extending to fields such as drug design, architecture, and engineering. These advancements represent an innovative pathway to tackling global challenges like climate change[4]. As enterprises increasingly incorporate sustainability considerations into their decision-making processes, the role of generative AI in supporting ESG (environmental, social, and governance) efforts is expected to grow, further reinforcing its value as a tool for sustainable development[8].
Challenges and Considerations
Generative AI offers transformative potential for a sustainable future, yet it presents several challenges and considerations that need careful attention. One of the primary concerns revolves around data privacy and security. The vast amounts of data consumed by generative AI systems can be inadequately governed, originating from sources that are potentially biased or used without consent, which can lead to biased outputs and privacy issues[9[10]. Furthermore, user data might be sold or given to third parties for marketing or surveillance, necessitating caution in sharing sensitive information with AI tools[5].
The environmental impact of AI technologies is another critical consideration. While generative AI can aid sustainable development, it also contributes to environmental challenges through its substantial computational power requirements, leading to increased carbon emissions and electronic waste[8]. Balancing the benefits of AI against its environmental footprint is crucial for its sustainable deployment.
From an ethical standpoint, generative AI poses significant risks. The automatic generation of content can perpetuate biases inherent in training data, resulting in harmful or biased language that requires human oversight to align with ethical business practices[10]. Moreover, the adoption of generative AI systems affects organizational structures and workforce dynamics, necessitating strategic planning to mitigate negative impacts while leveraging potential growth[9].
To navigate these challenges, it is essential to prioritize AI trust and transparency technologies. These solutions can enhance the governance of AI systems, ensuring ethical use and minimizing potential risks[2]. Additionally, conducting thorough assessments of AI models during the early stages of development can align their implementation with sustainability goals and ethical considerations[7] [11].
Case Studies
Generative AI has been increasingly applied in various domains to address sustainability challenges. In marketing, generative AI has been used to create creative content, such as personalized emails. This application has shown measurable outcomes, including reductions in the cost of generating content and increases in revenue due to the enhanced effectiveness of high-quality content at scale[12].
In the healthcare sector, the democratization and accessibility of generative AI tools have raised concerns about inadvertently disclosing sensitive information. For example, a medical researcher might unintentionally expose patient data, leading to breaches of trust and legal implications. Companies are recommended to implement clear guidelines and governance to safeguard sensitive information[9].
In the environmental domain, studies have highlighted the application of AI and machine learning in forestry, biodiversity research, and conservation. A comprehensive review identified 172 primary studies focusing on the use of AI in these areas, emphasizing the importance of innovative approaches to sustainability[3].
Future Prospects
The future of generative AI holds significant promise as a catalyst for sustainable development and business transformation. As these technologies become increasingly integrated into various sectors, their potential to enhance productivity and drive innovation becomes more apparent[13]. One key area of focus is the convergence of generative AI with other transformative technologies, such as those driving decarbonization efforts. This synergy could amplify the collective impact of these advancements, paving the way for a more sustainable future[7][1].
Generative AI's role in sustainability is not just a matter of technological innovation but also of strategic implementation. By promoting low-power electronics, efficiency-driven systems, and the creation of energy-efficient computer chips, generative AI can help reduce environmental impact[8]. Moreover, AI technologies can assist in sustainability and ESG (Environmental, Social, and Governance) efforts, providing a unique pathway to address environmental challenges through advanced technology[8][1].
However, it is important to consider the societal implications of generative AI. The potential for AI to enhance productivity could lead to the displacement of some jobs, necessitating a shift in workforce skills and roles[14]. Supporting workers in acquiring new skills is crucial for translating increased productivity into sustainable and inclusive economic growth[15].
Finally, the ethical, societal, and environmental implications of generative AI should be continuously assessed to ensure that these technologies contribute positively to achieving Sustainable Development Goals (SDGs)[11]. Emphasizing trust and strategic implementation will be critical in harnessing the full potential of generative AI for a sustainable future[7].
References
[1] Insights. (n.d.). Generative AI and Sustainable Development: Building a Greener Future. Insights. https://insightss.co/blogs/generative-ai-and-sustainable-development/
[2] Gartner. (n.d.). Gartner Experts Answer the Top Generative AI Questions for Your Enterprise. Gartner. https://www.gartner.com/en/topics/generative-ai
[3] Shivaprakash, K. N., Swami, N., Mysorekar, S., Arora, R., Gangadharan, A., Vohra, K., ... & Kiesecker, J. M. (2022). Potential for Artificial Intelligence (AI) and Machine Learning (ML) Applications in Biodiversity Conservation, Managing Forests, and Related Services in India. Sustainability, 14(12), 7154. https://doi.org/10.3390/su14127154
[4] Masterson, V. (2024, February 12). 9 ways AI is helping tackle climate change. World Economic Forum. https://www.weforum.org/stories/2024/02/ai-combat-climate-change/
[5] University of Alberta Libraries. (2024, October 31). Using generative AI. University of Alberta. https://guides.library.ualberta.ca/generative-ai/ethics
[6] Saenko, K., & The Conversation US. (2023, May 25). A Computer Scientist Breaks Down Generative AI's Hefty Carbon Footprint. Scientific American. https://www.scientificamerican.com/article/a-computer-scientist-breaks-down-generative-ais-hefty-carbon-footprint/
[7] PwC. (n.d.). How generative AI model training and deployment affects sustainability. PwC. https://www.pwc.com/us/en/tech-effect/emerging-tech/impacts-of-generative-ai-on-sustainability.html
[8] Pratt, M. K. (2023, October 20). Generative AI's sustainability problems explained. TechTarget. https://www.techtarget.com/sustainability/feature/Generative-AIs-sustainability-problems-explained
[9] Lawton, G. (2024, July 23). Generative AI ethics: 8 biggest concerns and risks. TechTarget. https://www.techtarget.com/searchenterpriseai/tip/Generative-AI-ethics-8-biggest-concerns
[10] Chugh, V. (2023, July 20). Ethics in Generative AI. DataCamp. https://www.datacamp.com/tutorial/ethics-in-generative-ai
[11] Vinuesa, R., Azizpour, H., Leite, I., Balaam, M., Dignum, V., Domisch, S., Felländer, A., Langhans, S. D., Tegmark, M., & Fuso Nerini, F. (2020). The role of artificial intelligence in achieving the Sustainable Development Goals. Nature Communications, 11, Article 233. https://doi.org/10.1038/s41467-019-14108-y
[12] Chui, M., Hazan, E., Roberts, R., Singla, A., Smaje, K., Sukharevsky, A., Yee, L., & Zemmel, R. (2023, June 14). The economic potential of generative AI: The next productivity frontier. McKinsey & Company. https://www.mckinsey.com/capabilities/mckinsey-digital/our-insights/the-economic-potential-of-generative-ai-the-next-productivity-frontier#introduction
[13] Zewe, A. (2023, November 29). What does the future hold for generative AI? MIT News. https://news.mit.edu/2023/what-does-future-hold-generative-ai-1129
[14] Elsevier. (2020). ScienceDirect. https://www.sciencedirect.com/
[15] McKinsey & Company. (2023, August 25). What's the future of generative AI? An early view in 15 charts. https://www.mckinsey.com/featured-insights/mckinsey-explainers/whats-the-future-of-generative-ai-an-early-view-in-15-charts
About the Author
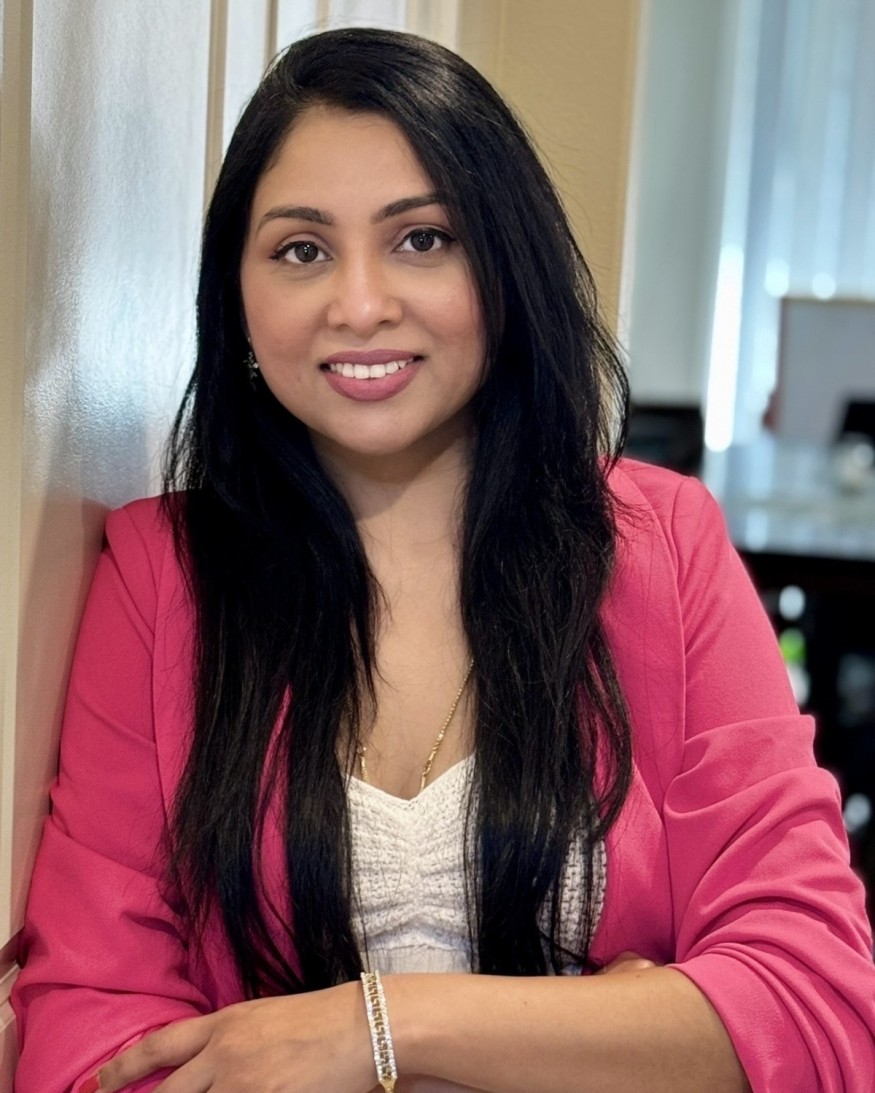
Neelam Koshiya, a Principal Solutions Architect and Generative AI Specialist at Amazon Web Services (AWS) leverages over 16 years of experience in cloud computing and machine learning to drive sustainable, AI-powered innovations. Known for her expertise in designing scalable AI solutions and her dedication to sustainability, Neelam explores the transformative role of generative AI in addressing environmental challenges. Her work emphasizes ethical AI development, energy efficiency, and responsible data practices, aligning advanced technology with the Sustainable Development Goals.