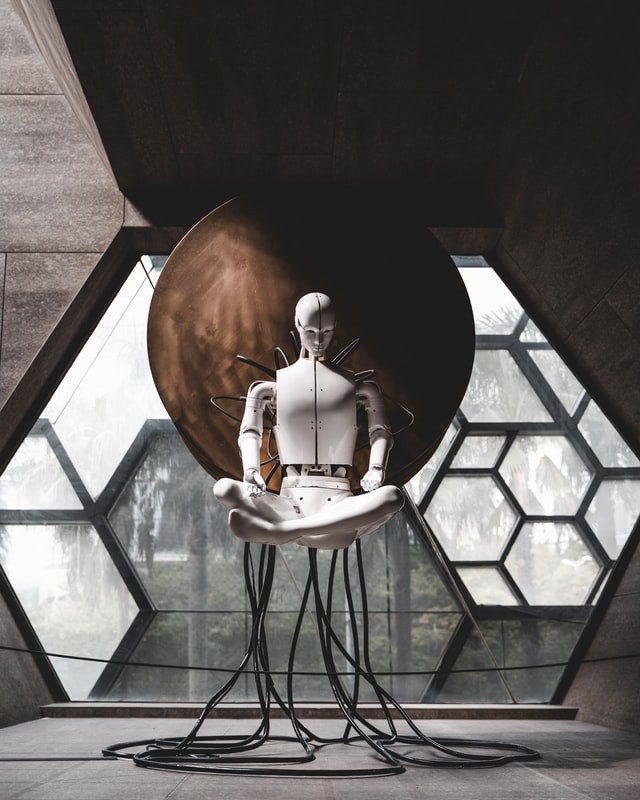
In hospitals, it helps doctors decipher X-rays, and in banks, it helps calculate financial risks. Artificial intelligence has been used in commercial relatively recently, but it is no less exciting. But how is make success in such an implementation? The answer is MLOps.
From 2012, when one researcher won a competition for image recognition using artificial intelligence, and to this day, when machine learning has become part of the technology industry, all experts understand that MLOps need for the successful use of AI.
Cloudera's data engineer, Santiago Giraldo, estimates that only 13% of all projects go through the experimental stage, while others don't. Іn other words, MLOps is a set of practices that guarantee that AI will not get into 87% of "defective" projects and will be successfully launched in your company.
MLOps was created as an analog of DevOps - a modern practice of creating, implementing, and running programs for corporations. Namely, the "teacher for AI" is the result of the fruitful collaboration between IT teams (Ops) and software developers. The large MLOps team includes scientists who curate the datasets and ML engineers who automatically manage the data.
The cybersecurity professor believes that MLOps is the result of the evolution of DevOps. While DevOps focuses on security and IT product management, MLOps focuses on the process of development. And such an emphasis has many advantages. Because, according to experts, the market for MLOps solutions will reach $ 4 billion in 5 years.
Projects using artificial intelligence undergo a large number of experiments, as a result of which they receive additional settings and retraining. Therefore, AI must have a strong infrastructure for MLOps, which can be scaled if the company grows. And this is a challenge for technology professionals in terms of implementation and management.
"Yes, it's true, MLOps needs a lot of infrastructures. But if you want to know how effective your project is, its monitoring has to be multifaceted to look at the project from different perspectives. And that requires a lot of resources." - says the analyst strategist at SAS.
Most modern tools have a limited view of the data, which is why there is a need to test AI projects. You need to constantly test the project, perform calibrations if necessary, otherwise your project may cease to benefit the company, and in some cases even begin to cause harm.
RapidMiner's vice president of customer success believes that MLOps is an indispensable assistant in commercial projects. If as a result of monitoring you see that performance indicators are falling, then a set of MLOps practices will help you to intervene in the project. This will save you millions of dollars on your budget.
"To make sure that machines have a positive impact on business, they need to be monitored and maintained. Then we can be sure that they can move to new data without harming the business. We often hope that machines can work forever. , but, unfortunately, this is not true "- says the vice president of customer success at RapidMiner.
Finally, the undoubted advantage of using MLOps during a coronavirus pandemic is that the AI will change. Many models of artificial intelligence have become inoperable only because they have not received up-to-date data sets. And MLOps can provide up-to-date updates that will allow many projects to continue to exist.
Well, it seems that the maturity of the practices used by the "teacher" of artificial intelligence is universal and can be used in a wide range. MLOps is accustomed to modern business requirements and is an indispensable helper in the context of the COVID - 19 pandemic.
© 2025 ScienceTimes.com All rights reserved. Do not reproduce without permission. The window to the world of Science Times.