Reinforcement or reward learning is a term used in operant conditioning. According to Very Well Mind, it refers to a stimulus that increases the likelihood of a response to occur.
This is usually used in the field of education or learning to teach kids in school or introduce new sets of behavior to an individual that is rewarding.
But researchers from Texas A&M University have found a new way to use it on an algorithm. This reinforcement-based algorithm automates the process of predicting the properties of the environment beneath the ground to forecast natural reserves, like oil and gas, accurately.
The researchers said that the algorithm is designed to the principles of positive reinforcement. The computer algorithm gets rewards for every accurate prediction it makes on the description of the underground environment, like the pressure and the flow expected from boreholes.
According to associate professor Siddharth Misra, the underground environment's depth is completely opaque that makes it hard to see that is why instruments that measure pressure and rates of flow are being used.
He added that the current study is just one step to have an automated way of using information that accurately characterizes the properties underground. The full findings of the study are published in the journal Applied Energy's December issue.
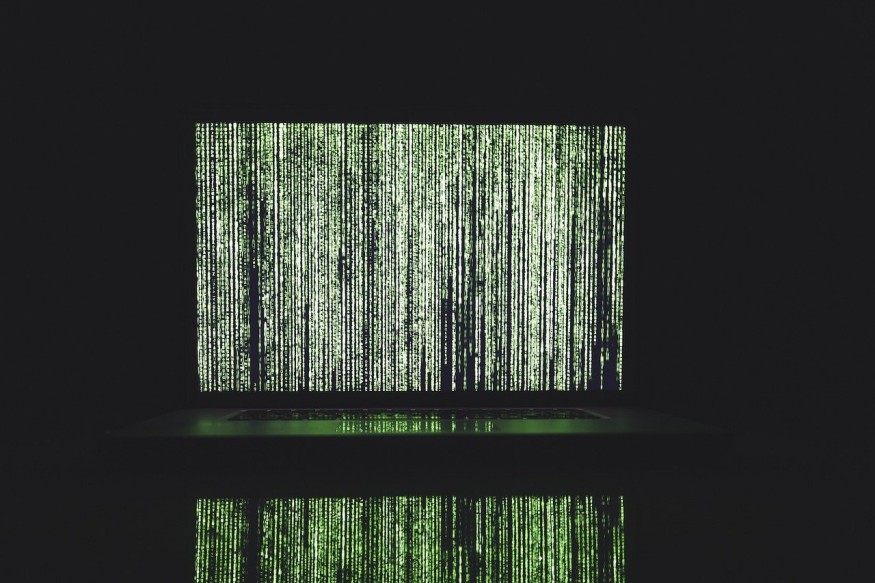
Automated Forecasting of Oil and Gas Reserves
In a press release of Texas A&M University, they said that the reinforcement-based algorithm could greatly facilitate forecasting oil and gas reserves to predict groundwater systems and anticipate seismic hazards.
Drilling operators could exactly know the pressures and flow rates of the liquid or gas in the boreholes by placing sensors that are plugged into mathematical models that predict properties of porosity and permeability of the rocks.
These mathematical models used to require extensive human intervention and give a flawed picture of the underground environment.
Another advantage of using the reinforcement-based algorithm is that it does not make presuppositions about the pattern of the data, according to TechXplore. This makes it less biased and reduces the chances of committing errors in predicting the underground environment.
How Does Reinforcement Work On the Algorithm?
Misra and his team, who developed the reinforcement-based algorithm, explained that the algorithm learns to make a series of decisions based on its computational environment's response.
They receive rewards for favorable predictions and are punished for unfavorable ones. Over time, they arrive at the correct solution as they maximize their accrued reward. Therefore, they accurately predict the oil and gas reserves in the underground environment.
The algorithm first randomly guesses a value for the porosity and permeability of the rocks in the subterranean surface then calculates the flow rate and pressure it expects from the borehole. If it fails to match the historical data, the algorithm is penalized and forced to correct its next guess. But if it is correct, it further guesses that direction.
Check out more news and information on Algorithm on Science Times.