Scientists are now integrating gait data and machine learning to advance the tools used to monitor and predict the progression of multiple sclerosis (MS).
According to a Medical Xpress report, monitoring the development of gait issues related to MS can be challenging in adults aged above 50 years old, necessitating a clinician to differentiate between issues associated with MS and other age-related problems.
New research of this approach authored by University of Illinois Urbana Campaign's graduate student Rachneet Kaur; kinesiology and community health professor Manuel Hernandez; and industrial enterprise engineering and mathematics professor Richard Sowers, was published in the Institute of Electric and Electronics Engineers Transactions on Biomedical Engineering journal. (Predicting Multiple Sclerosis from Gait Dynamics Using an Instrumented Treadmill - A Machine Learning Approach)
As indicated in the report, MS can show itself in various ways in the roughly two million people it's affecting worldwide and walking problems are a common symptom.
According to the said research, about 50 percent of the patients need assistance in walking within 15 years of the onset of the disease.
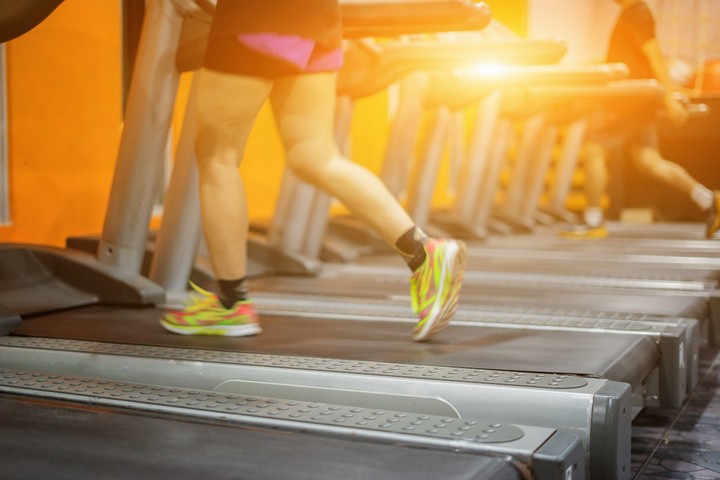
Machine-Learning Techniques
Commenting on their work, Hernandez said they wanted to get a sense of the interactions between aging and concurrent changes related to MS disease, and whether they can differentiate between the two older adults.
The kinesiology and community health professor added machine-learning techniques that appear to be effective at spotting multifaceted unseen changes in performance.
The study authors hypothesized that these analysis methods might also be helpful in predicting instantaneous gait changes in individuals who have MS, Hernandez explained.
Through the use of an instrumental treadmill, the research team gathered gait data, normalized size of the body, as well as demographics, from 20 adults who have MS and 20 age-, height, gender, and weight-matched elderly without the disease.
The participants were observed while walking at a comfortable pace for a maximum of 75 seconds while specialized software caught gate events, equivalent ground reaction forces, and center-of-pressure position during every walk.
Variations in Gait Analyzed
The research team extracted characteristic spatial, kinetic, and temporal features of each participant in their paces to analyze variations in gait during every trial.
Changes in different gait features, which include a data feature also known as the butterfly diagram, helped the researchers identify differences in gait patterns between volunteers
The diagram got its name from the butterfly-shaped curve developed from recurring center-of-pressure trajectory for multiple continuous paces during a walk of a subject and is linked to the crucial neurological functions, according to the study.
Kaur explained, they study the effectiveness of a machine-learning framework that's gait dynamics-based, to categorize strides of older people who have MS from healthy controls to generalize through different walking tasks, not to mention, over new subjects.
This proposed approach, Kaur said, is an advancement toward the development of assessing markers for medical professionals so they can predict elderly with MS who are likely to experience "a worsening of symptoms in the near term."
Thorough Investigations from Future Studies
Meanwhile, Sowers said, future studies can offer more thorough investigations to manage the small cohort size of the study.
He added Biomechanical systems like walking, for one, are poorly modeled systems, making it a struggle to identify problems in a clinical background.
In this particular research, the study authors are attempting to extract conclusions from sets of data that comprise a lot of measurements from every individual, although a smaller number of these individuals.
The findings of this study make substantial progress in the area of clinical machine learning-based disease-prediction approaches.
A related report is shown on Mission Gait's YouTube video below:
RELATED ARTICLE: Multiple Sclerosis: Stem Cell Transplant Treatment, Principle, Risks
Check out more news and information on Medicine & Health on Science Times.