Neural networks are one of the trending technology today. It is sometimes called artificial or simulated neural networks. This complex technology is among the greatest chunks of machine learning that makes artificial intelligence work as programmed.
In recent advancements, neural networks have been a part of numerous studies regarding drug-target interactions and complicated chemical reactions. New research from MIT experts attempted to solve the biggest problem of neural networks in molecular simulations.
Importance of Neural Networks in Molecular Energy Simulations
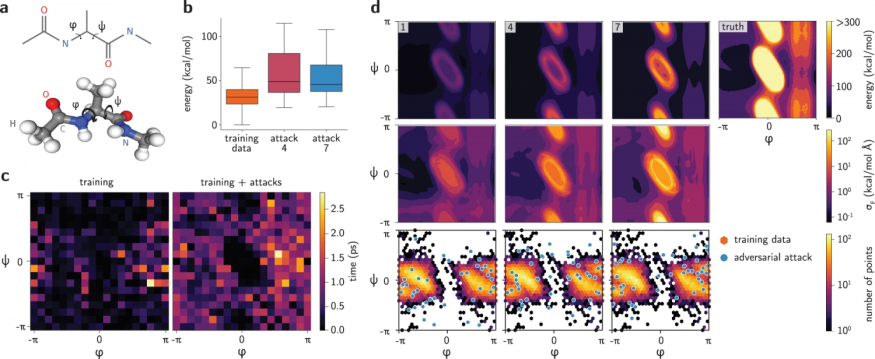
Some regard neural networks as a faster choice than other simulations, such as the quantum mechanics approach. However, the great features of the neural network come at a staggering price in the form of reliability. Most of the machine learning technology in the present time is still under development, and there is no confirmed neural network that could exceed the current standards accepted.
Machine learning usually fails, and the intermittent reliability of the technology is evident in most recent studies that attempt to overclock either AI-powered algorithms or quantum computers.
With that said, experts at the Massachusetts Institute of Technology conducted research to differentiate the potential limits and exceed the capacity of neural networks to help machine learning set the right expectations.
Differentiating the limits of neural networks is also essential in predicting the mapping of structures, also known as potential energy surfaces or PES. This type of surface is comprised of molecular configurations located at the chasms or quantum mechanical points near the atoms.
MIT's recent study presents a potential solution through a novel method to distinguish the neural networks' safe zones. According to MarkTechPost, the method is in the form of an adversarial attack towards the molecules previously studied along with other classes of problems.
MIT experts utilized their very own Gomez-Bombarelli laboratory to develop the first-principle simulation that could be fused with machine learning in an attempt to speed up the process and exceed that normal capacity of simulations.
The normal process of machine learning in its actual simulations is only possible through conducting examinations over molecules.After the simulation phase, the molecule fractions are then transferred to neural networks to process predictions required for any investigation, such as fusion of catalyst to water for hydrogen extraction, construction of cheaper polymer electrolytes for energy-efficient vehicles, and many more.
Zeolites Provide More Accurate Prediction
The only problem neural networks experience is that their capability is limited based on the data they were trained with. To solve this conundrum, running the data across different neural networks was considered to predict the energy surface precisely.
The only downfall this method will incur is that the uncertainties in the prediction could cause a significant discrepancy in the outcomes.
The new study suggests additional methods in predicting supramolecular interactions through zeolites. These materials are composed of cavernous crystals which serve as molecular sieves. Through zeolites, there is a chance that the selectivity in molecular complexities will be fast and accurate.
According to the study, Zeolites could be the potential solution to machine learning predictions and simulations, provided that the material is present in a large structural form.
This was proven when 15,000 examples were trained to the neural network, resulting in an 80% simulation success rate. In addition, another 5,000 new data sets were trained, resulting in the neural network performing at a stunning 92% success rate.
The examination coverage was published in the journal Nature Communications, titled "Differentiable Sampling of Molecular Geometries With Uncertainty-Based Adversarial Attacks."
RELATED ARTICLE : How To Know If Your Cat Is Happy? AI-Powered App Uses Camera To Tell Feline's Mood
Check out more news and information on Artificial Intelligence in Science Times.