Researchers at Universität Hamburg have recently developed a new technique to teach robots to grasp objects and manipulate them using the multi-fingered robotic hand.
In recent years, as specified in a Tech Xplore report, robotics has developed growingly advanced robotic systems, many of which have artificial hands or robot hands that have multiple fingers.
To complete daily tasks in both public settings and homes, there is a need for robots to be able to use their hands to grasp and maneuver objects effectively.
Enabling dexterous manipulation that involves multiple fingers in robots, though, has thus far proven challenging. This is mainly because it is an advanced skill that encompasses adjusting objects' shape, configuration, and shape.
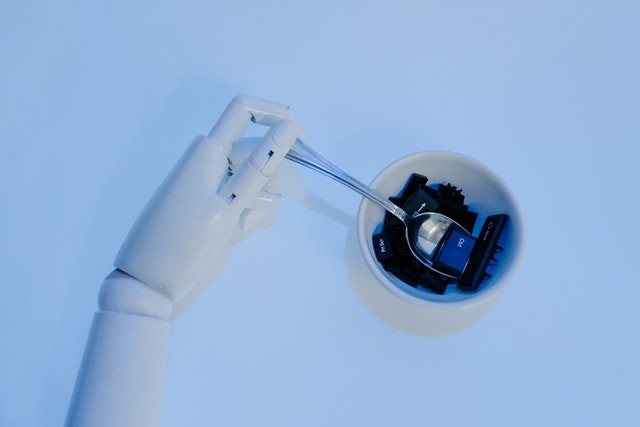
'Teleoperation System'
This new technique, reported in the IEEE Transactions on Neural Networks and Learning Systems journal, enables a robotic hand to learn from humans through teleoperation and adjust its manipulation tactics based on human hand postures and the data collected when interacting with the environment.
According to one of the researchers Dr. Chao Zeng, who conducted the study said the original notion behind this study was to develop a teleoperation system that can transfer the manipulation skills of a human hand to a multifigured robot hand "so that human user can teach a robot hand" to carry out tasks online.
Zheng added, that there are two basic objectives of this work. First, unlike other state-of-the-art approaches, one wouldn't want to wear a glove "with optical markers on it."
Zheng and his colleagues wanted their robot to obtain dexterous manipulation skills by observing human demonstrations.
Dexterous Manipulation
In this study, instead of forcing human users who train the robot to wear gloves with optical markers, as done in other previous research, the team wanted the user to be able to move his fingers freely without any physical limitations.
They also used cameras instead to capture images of the hand postures of human users. This proved to be quite a challenge, although they eventually obtained promising results.
Explaining the second objective, Zeng said it was to use the robotic hand to obtain compliant behaviors, as humans do, so it would be able to face physical contract-rich interaction tasks with anticipated dexterity.
Deep Neural Network
In this study, explained Zeng, they also wanted to adopt force control on the robot hand. Nevertheless, directly training a so-called deep neural network or DNN to produce the desired force control commands for a robot at run time is quite challenging. To solve the issue, the team took a two-step approach.
The first-step approach developed by Zeng and his team encompassed capturing the human users' posture and mapping this onto the robot's joint angles using a DNN. After the training, it could effectively examine images of the hands of a human hand and produce matching joint angles for the hands of the robot.
A new approach to enhance multi-fingered robot hand manipulation - News Update #roboticsainews #robotics #robots #ai #artificialintelligence #digitaltransformation #technology #futurework #engineering #automation https://t.co/hXrNDhXq6o
— RoboticsAINews (@RoboticsAINews) July 12, 2022
As a second step, Zeng continued explaining that they designed a force control technique to predict the desired force commands at each time step given the present reference angle, a similar News Update report said.
As explained by the researcher, these two components of the approach can be seamlessly incorporated into the teleoperation system to enhance the compliance of the robotic hand, as the team had set out to do.
Related information about robot hand manipulation is shown on Shadow Robot's YouTube video below:
Check out more news and information on Robots in Science Times.