According to a peer-reviewed study published in Remote Sensing in May, Israeli researchers identified a mechanism to predict earthquakes 48 hours ahead with 80% accuracy. The Ariel University and Center for Research & Development research team were able to examine potential precursors to several big earthquakes that happened in the last 20 years by examining changes in the Earth's ionosphere, the sliver of atmosphere that meets the vacuum of space.
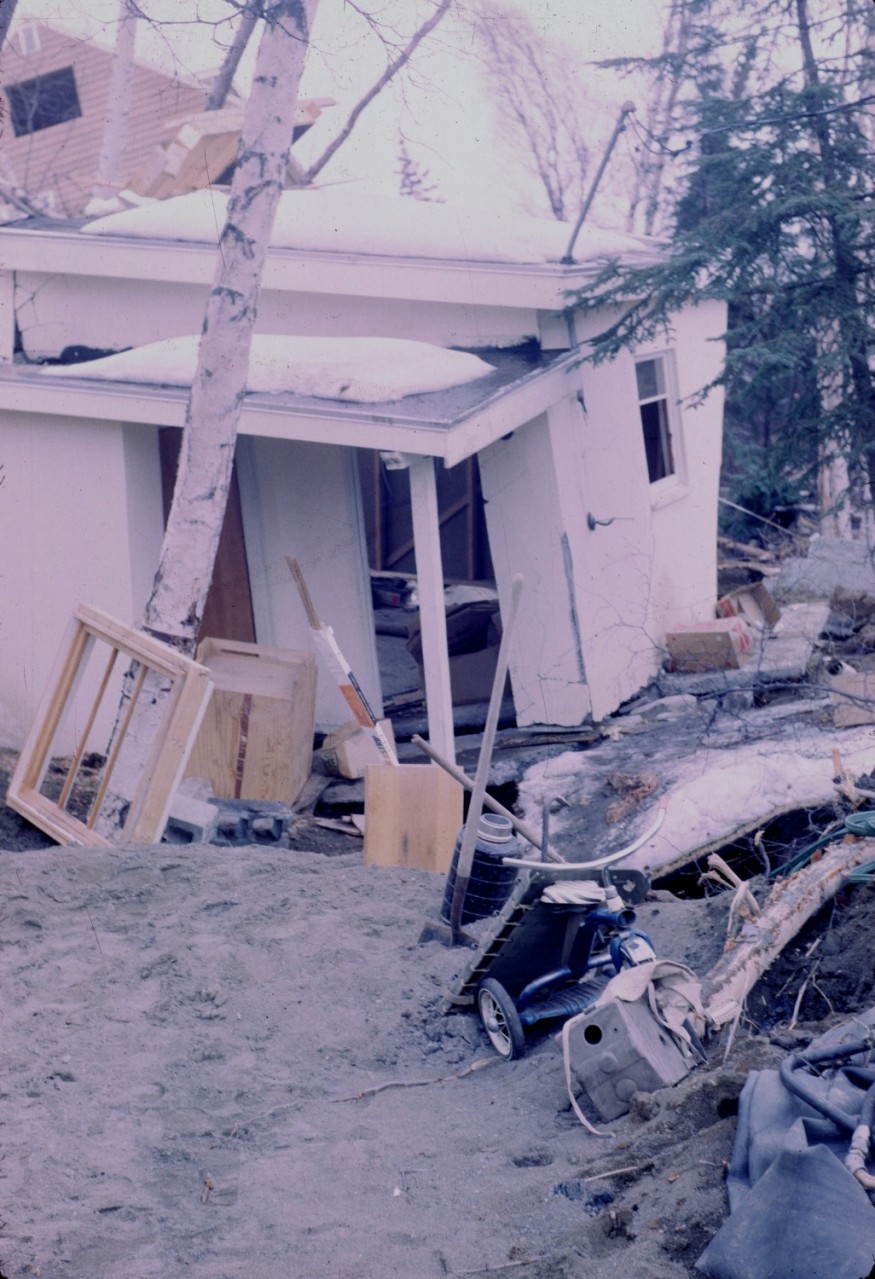
Research Summary on Earthquake Prediction
The researchers categorized large earthquakes as those that exceeded Mw 6 on the Moment magnitude scale, which quantifies the magnitude of an earthquake based on its seismic movement. The team's solution involved implementing a machine learning support vector machine (SVM) algorithm and using GPS map data of ionospheric total electron content to determine electron charge density. Using this technique, they discovered that an earthquake might be correctly predicted with an accuracy of 80%. Furthermore, the researchers could forecast when an earthquake will not occur in a certain area with 85.7 percent accuracy.
Datasets and Methodology of Earthquake Prediction
The main goal of the research was to learn more about the possibility of predicting earthquakes using TEC value anomalies. The researchers removed all earthquake events during elevated solar activity to eliminate all non-related geodynamic impacts such as solar flares, coronal mass ejections, geomagnetic storms, and X-ray flux events.
The ionosphere is a region of the Earth's upper atmosphere that extends (roughly) between 60 and 1000 km, where extreme high-energy ultraviolet (EUV) and solar X-ray radiation ionizes atmospheric atoms and molecules, generating a layer of free electrons. GNSS technology provides a one-of-a-kind tool for global monitoring of the ionosphere's state. It allows for calculating the total electron content (TEC) of the global ionosphere/plasmasphere up to a height of about 20,000 km.
The researchers also used the US Geological Survey (USGS) earthquake dataset, which offers the most rapid and precise information about the location, size, and depth of all documented earthquake events worldwide. On the other hand, the International GNSS Service (IGS) Analysis Centre dataset was used for the TEC dataset, which contains final global ionospheric TEC maps extracted from averaging four different available models. The IGS collection also provides rapid global TEC maps in cylindrical format, RMSE values, and rapid TEC maps in polar projection.
NOAA SWPC data were used for the solar flare and geomagnetic datasets, which provide real-time and archived auroral and solar activity data since June 1996. It is based on the space environment monitor instrument subsystem carried on board Geostationary Operational Environmental Satellites. The collection includes the strongest 50 solar flares each year and the specific magnitude, place, and time of occurrence for each flare. The dataset contained 1250 solar flares.
The overall methodology began with a TEC data removal approach, followed by preprocessing and data splitting. Finally, the researchers attempted to forecast earthquakes using SVM and preprocessed ionospheric VTEC data.
SVM is a supervised machine learning strategy based on the kernel method commonly used for classification and regression tasks. The researchers attempted to optimize the SVM hyperparameters to identify the kernel function and parameters that produced the most accurate model. The goal of Bayesian optimization is to collect observations that reveal as much information about the function as possible, including its optimum location.
The researchers used a polynomial kernel function with a degree of two and the Bayesian optimization algorithm to obtain the best hyperparameters possible in the shortest computation time, resulting in an accuracy of 82.5 percent, precision of 85 percent, recall of 80 percent, and 65.7 percent in both HSS and TSS skill scores.
RELATED ARTICLE : Earth's Outer Core Is Changing Based on Seismic Waves From Earthquakes, Study Claims
Check out more news and information on Technology in Science Times.