Researchers from the Mahmood Lab at Brigham and Women's Hospital have created a deep learning system that can teach itself to learn features. The system aims to detect related cases in a huge pathology image library.
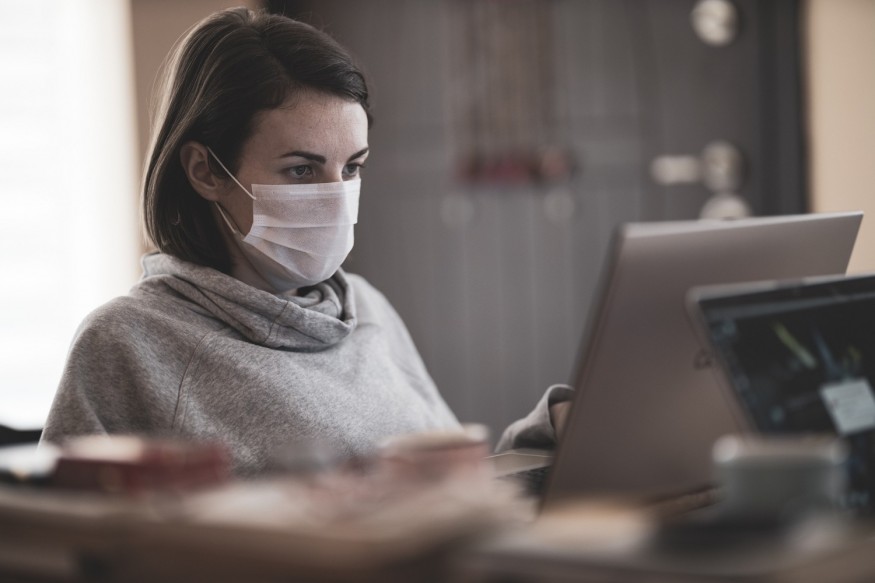
Self-Supervised Image Search for Histology
The new technology is called SISH (Self-Supervised Image Search for Histology). It functions as a pathologic picture search engine. It offers a wide range of possible uses, including detecting rare diseases. Additionally, it would assist doctors in identifying which individuals are most likely to respond to similar treatments. The paper is published in Nature Biomedical Engineering.
Faisal Mahmood, Ph.D., Senior Author of Brigham's Department of Pathology, said that the algorithm could help detect rare diseases and locate instances with comparable morphologic patterns without requiring manual annotations or sizable datasets for supervised training. The method may enhance pathology training, illness subtyping, tumor diagnosis, and identification of unusual morphologies.
SISH Can Handle Large Size Data
An enormous quantity of digital information and reference photos can be stored in modern electronic databases, especially in pathology, through whole slide images (WSIs). However, because each WSI is gigapixel-sized and there are so many photos in huge libraries, searching for and obtaining WSIs can be time-consuming and difficult. Scalability continues to be a significant barrier to effective utilization as a result.
On the other hand, SISH educates itself to learn feature representations that can be used to discover cases with equivalent features in pathology at a consistent pace, regardless of the size of the database.
SISH Can Find Data at a Fast Speed
The researchers evaluated the efficiency and capacity of SISH to gather information on interpretable disease subtypes for both common and rare cancers. The library of thousands of entire slide images from more than 22,000 patient cases, with more than 50 different disease kinds and more than a dozen anatomical sites, was successfully searched by the algorithm with speed and precision.
In several situations, including the retrieval of illness subtypes, the speed of retrieval exceeded alternative techniques, especially as the size of the image database grew to include thousands of images. SISH could keep up a steady search speed even as the repositories grew in size.
ALSO READ: How AI Deep Learning Neural Networks Prevent Species Extinction and Protect Indigenous People
SISH Drawbacks
The method has certain drawbacks, such as a high memory requirement, a lack of context awareness in big tissue slides, and the fact that it is restricted to a single imaging modality.
Yet, the method showed that it could efficiently retrieve photos from various datasets, regardless of the size of the repository. Additionally, it showed that it was adept at diagnosing specific rare disease types and could act as a search engine by identifying specific areas of photos that would be important for diagnosis. This work could considerably improve the diagnosis, prognosis, and analysis of future diseases.
According to News Medical Life Sciences, Mahmood said they anticipate SISH to help facilitate illness identification as picture database sizes continue to increase. They consider multimodal case retrieval, which involves searching for related patient cases utilizing data from pathology, radiology, genomics, and electronic medical records, to be an important future approach in this field.
Check out more news and information on Technology in Science Times.