There is a lot of buzz in the media about chatbots like GPT-3, and even if you're not interested, someone may still ask you about it. However, the general public doesn't fully understand these systems. To get a better grasp of what's really going on, you could turn to experts like Stanford Professor Christopher Potts. He has a deep understanding of how chatbots work, as demonstrated in a recent video.
One interesting observation he makes is that different chatbots can give different answers to the same question. While this may be entertaining as a demo, it becomes problematic when used as a search engine, as incorrect answers won't be as easily recognized as erroneous. It's similar to using a product like Grammarly or a spell checker - it offers suggestions, but you must verify that they are correct. It's rare, but possible to get the wrong suggestion, as reported by Hackaday.
Chatbot's Language Processing
Technically speaking, the architecture of these chatbots relies on a component called "the transformer," which analyzes the input words and their positions. This concept originated from Google in a 2017 paper and has greatly impacted language processing, leading to the development of chatbots like GPT-3. As Professor Potts explains, the sophisticated algorithm is made up of straightforward components, yet its exceptional performance is not fully understood. The full hour-long lecture provides even more insights and is worth watching if you're interested in this cutting-edge technology.
Just like with any advanced technology, you don't have to have a complete understanding of it to use it. However, there are potential issues such as producing incorrect results or copying sensitive information. It's uncertain where this technology will lead, but it is certainly advancing. While we may not fully comprehend chatbots at present, it's also true that they don't fully comprehend us.
Users may have noticed that chatbots are not the best at having conversations. While there are some exceptions such as Microsoft's Xiaoice in China and Alibaba's Dian Xiaomi, most chatbots struggle to hold human attention for more than 15 minutes. Despite this, the adoption of chatbots is on the rise and is predicted to power 85% of all customer service interactions by 2020. However, there is hope for improving the conversational abilities of chatbots. Researchers from Facebook's AI Research and Stanford University have recently published a paper on a chatbot that can improve itself by learning from conversations.
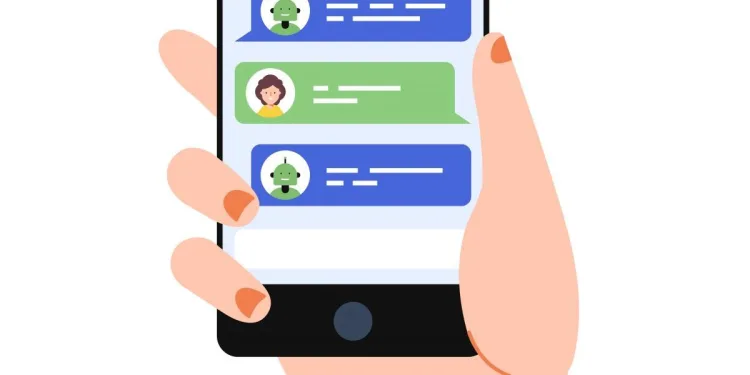
Self-Training Systems
According to a recent paper in 2019 by researchers from Facebook's AI Research and Stanford University, chatbots have the potential to improve their conversational abilities through self-learning. If the conversation is going well, the user's responses can serve as new training data for the chatbot to imitate. When the chatbot believes it has made a mistake, it can ask for feedback, which helps improve its dialogue abilities. This new training process does not require any special structure, numerical feedback, or human intervention, as reported by VentureBeat.
However, there is a risk that chatbots may reinforce errors in their self-training, leading to bizarre conversations. The researchers solved the potential problem of chatbots reinforcing errors by using satisfaction. They had contract workers rate the quality of the AI agent's responses on a scale from 1 to 5 to create a "satisfaction" dataset. This was used to teach the system to predict whether a human response was satisfied or unsatisfied.
As the chatbot interacted with a human, it trained on two tasks at the same time: dialog and feedback. After each exchange, the chatbot took into account prior conversations to generate its next reply and a satisfaction score. If the score reached a certain threshold, the chatbot would extract training data from the previous context and the human response. But if the score was low, the chatbot would request feedback with a question and use the response as a new example for the feedback task.
RELATED ARTICLE : Anthropic's AI System Claude Tweaks OpenAI's ChatGPT to Align It With Human Intentions Thru Its 'Constitutional AI'
Check out more news and information on Technology in Science Times.