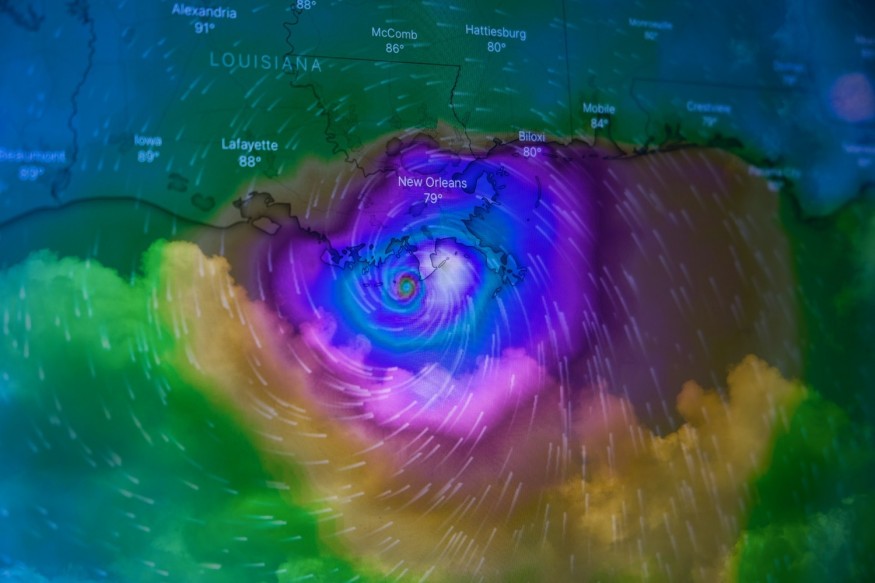
Imagine a scenario where a bustling coastal city is preparing for an impending storm. The stakes are high, and the accuracy of weather forecasts can be the difference between timely evacuations and potential disasters. In such critical moments, the fusion of Artificial Intelligence (AI) and neural networks emerges as a beacon of hope, offering a glimpse into a future where weather predictions are more accurate and driven by cutting-edge technology.
The realm of weather modeling insights has witnessed a remarkable evolution over the years, propelled by the relentless march of technological advancements. AI and neural networks are at the forefront of this revolution, two potent tools that have redefined how we anticipate and respond to atmospheric changes. This blog post embarks on a journey through these digital corridors, exploring the transformative impact of AI and neural networks on the intricate art of weather forecasting.
Understanding AI and Neural Networks:
Artificial Intelligence (AI) and Neural Networks have emerged as game-changers in the realm of technology, reshaping our approach to intricate challenges like weather forecasting. AI embodies the capacity of machines to mimic human intelligence, enabling them to learn from data, recognize patterns, and make well-informed decisions. On the other hand, Neural Networks take inspiration from the human brain's structure, employing interconnected layers of artificial neurons to process information and derive meaningful insights.
Think of AI as a digital brain that rapidly and accurately analyzes colossal datasets, uncovering patterns and correlations that might elude human observation. Similarly, Neural Networks, under the AI umbrella, emulate our brain's information processing mechanism. Comprising layers of artificial neurons, they specialize in extracting distinct features from data and adjusting interconnected weights to identify complex patterns. This synergy of AI and Neural Networks equips weather forecasters with the tools to navigate atmospheric dynamics, enabling swift data processing and unveiling intricate trends that enhance the precision of weather predictions.
How Artificial Intelligence Improves Weather Forecasting
In the age of technological marvels, Artificial Intelligence (AI) emerges as a pivotal force in weather forecasting, substantially elevating the precision of predictions. Here's how AI is transforming the landscape:
Data Analysis Evolution: Gone are the days of relying solely on historical data. AI-driven algorithms now dissect immense datasets collected from satellites and global weather stations, detecting patterns that might elude human scrutiny due to data volume.
Incorporating Complex Physics: AI-powered computer models transcend traditional boundaries by incorporating intricate, atmospheric physics principles. Beyond predicting temperature, these models delve into storm severity and cloud formation processes, amplifying predictive capabilities.
Real-Time Ocean Insights: AI harnesses real-time ocean current mapping, amassing additional data points inaccessible via historical records alone. These insights enrich prediction accuracy significantly when dynamic conditions influence weather patterns.
Machine Learning Mastery: AI systems master meteorology through machine learning techniques like deep and reinforcement learning. The synergy of these methods enhances efficiency, hastens processing, and heightens precision, even when handling massive datasets.
Customized Localization: The essence of AI lies in personalization. AI-powered systems provide tailored predictions that cater to localized and specific weather information requirements, ensuring relevance and accuracy.
Challenges and Limitations:
While the fusion of Artificial Intelligence (AI) and weather forecasting holds remarkable potential, it has challenges and limitations. Let's delve into some key considerations:
Data Quality: AI thrives on quality data, and inaccurate or incomplete inputs can skew predictions. Ensuring data accuracy and reliability remains a persistent challenge.
Interpretability: AI models often produce accurate results, but understanding the rationale behind these predictions can be complex. Interpreting AI-generated forecasts can take time, leading to concerns about accountability and trust.
Bias and Generalization: AI models might inadvertently learn tendencies in the training data, leading to skewed predictions. Ensuring fair and unbiased forecasts across diverse regions and populations remains a critical challenge.
Data Overload: While AI's strength lies in handling vast datasets, too much data can overwhelm models, leading to slower processing times and potential accuracy compromises.
Dependency on Training: AI models heavily rely on prior training data. Rapidly evolving weather patterns or unprecedented events can challenge the accuracy of AI forecasts.
Conclusion
The collaboration between Artificial Intelligence and neural networks has transformed weather forecasting. These technologies now provide more dependable predictions about local climate changes. Meteorologists use real-time observations and intelligent connections between different weather factors to create accurate forecasts, making errors less likely.
These tools don't just help predict things like storm strength and duration. They also tackle more considerable challenges, like understanding the changing climate. This exciting progress means that meteorology's future looks bright. With these new techniques, we can expect forecasts to be more precise, efficient, and helpful, ushering in a new era of reliable weather predictions that benefit us all.