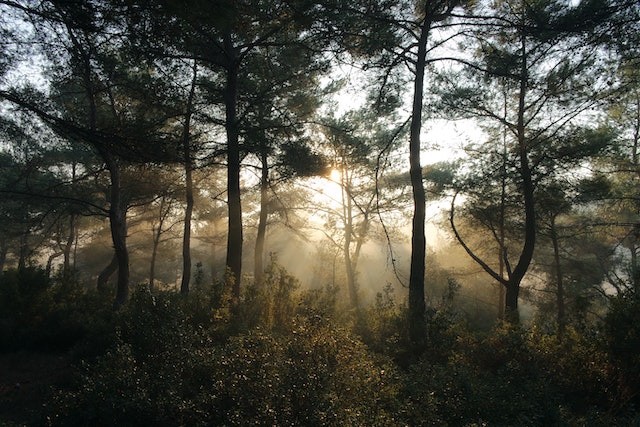
Artificial Intelligence (AI) has several applications. It was recently used to generate a map of trees and renewable energy projects, and the result was much clearer than the satellite imagery.
AI-Generated Map Better Than Satellite Imagery
Today, a first-of-its-kind map that employs generative AI to improve photographs taken from space was released. It shows the coverage of trees and renewable energy installations worldwide.
All of this is a component of a brand-new tool called Satlas from the Allen Institute for AI, established by Paul Allen, a co-founder of Microsoft.
It uses Sentinel-2 satellite imagery from the European Space Agency (ESA). It employs deep learning models to fill in features, such as what buildings could look like, to generate high-resolution photos. This feature is referred to as "Super-Resolution."
For the time being, Satlas concentrates on global tree cover and sustainable energy initiatives. Sentinel-2-monitored regions of the earth are featured in the data and updated monthly. The majority of the world is included, except for parts of Antarctica and open oceans.
It displays the onshore and offshore wind turbines and solar farms. It can also be used to track historical changes in the amount of tree canopy.
Those are crucial findings for politicians working to achieve climate change and other environmental goals. The Allen Institute claims there has never been a public tool this comprehensive and free. According to the map's creators, this is probably one of the earliest examples of super-resolution in a global map.
The Allen Institute team had to spend countless hours manually labeling 36,000 wind turbines, 7,000 offshore platforms, 4,000 solar farms, and 3,000 tree cover percentages to create Satlas. They developed the deep learning models in this manner, teaching them to recognize certain traits independently.
They fed the models many low-resolution photos of the same location captured at various times to achieve super-resolution. In order to forecast sub-pixel features in the high-resolution photos it creates, the model uses those images.
The Allen Institute intends to broaden Satlas to offer additional map types, such as one that shows the different crops grown worldwide.
Satlas Tool Warning
Although Satlas produces better imagery than those taken from satellites, it still has the same limitations as other generative AI models. According to experts, it is prone to "hallucinations."
According to Ani Kembhavi, senior director of computer vision at the Allen Institute, it drew structures in odd ways, which you can either term hallucination or poor accuracy. The building may be rectangular, but the model may have misinterpreted it as trapezoidal or another shape.
That might be because the model isn't very good at anticipating differences in regional architecture. Placing vehicles where the model believes they should be based on the training images is another classic example of hallucination.
The famous AI bot ChatGPT also hallucinates. According to one study, most of its responses, about 98%, to cancer-related questions included at least one treatment strategy that complied with NCCN guidelines. However, 34% of these comments had one or more contrarian suggestions, and 12.5% completely deviated from NCCN guidelines.
Check out more news and information on AI and GPT-3 in Science Times.