Abstract: Predictive models have emerged as essential tools in modern supply chain and logistics management, leveraging advanced algorithms and data analytics to enhance delivery accuracy and operational efficiency. By integrating machine learning techniques such as logistic regression, time series analysis, and decision trees, these models enable companies to forecast demand, optimize inventory, and mitigate disruptions. This article explores the transformative role of predictive models in logistics, highlighting their applications in inventory management, route optimization, and real-time decision-making. Real-world implementations demonstrate their ability to improve delivery accuracy by up to 80%, generate significant cost savings, and enhance customer satisfaction. Despite these benefits, challenges such as data quality issues and organizational resistance persist. Addressing these challenges and embracing future advancements, including IoT integration and sustainability initiatives, will further revolutionize supply chain ecosystems. This article provides actionable insights into the development, scaling, and business impact of predictive models, emphasizing their pivotal role in creating resilient and adaptive supply chains.
Keywords: predictive models, supply chain management, logistics, machine learning, delivery accuracy, operational efficiency, demand forecasting, inventory optimization, route optimization, data analytics, business impact, real-time decision-making, IoT integration, sustainability, adaptive supply chains
Predictive models have become an integral component in the evolution of supply chain and logistics management, harnessing the power of advanced algorithms and data analytics to boost operational efficiency and delivery accuracy[1]. These models utilize machine learning techniques to analyze large datasets, enabling companies to forecast demand, optimize inventory, and anticipate potential disruptions[2]. The strategic implementation of predictive analytics not only enhances logistical operations but also leads to substantial cost savings and improved customer satisfaction[3]. Notably, predictive models can achieve delivery accuracy rates as high as 80%, significantly impacting revenue and providing a competitive edge in the market[4].
Incorporating machine learning within predictive models allows for dynamic adjustments to changing market conditions, ensuring continuous accuracy in predictions. Techniques such as logistic regression, time series analysis, and decision trees are employed to refine these models, making them essential for intelligent data analysis and informed decision-making[5]. The development and scaling of these models pose challenges, particularly in terms of data quality and integration, but overcoming these hurdles unlocks transformative benefits across supply chain ecosystems[6].
Real-world applications of predictive models in logistics demonstrate their effectiveness in optimizing delivery routes, managing resources efficiently, and enhancing supply chain visibility[7]. By analyzing historical and multicarrier shipment data, some companies have successfully deployed predictive models that not only increase delivery accuracy but also generate annual revenue gains of up to $1 million[8]. This integration of predictive analytics facilitates proactive management of logistics operations, mitigating risks and ensuring seamless supply chain performance[9].
Despite the notable advantages, the deployment of predictive models also involves addressing controversies related to data privacy and organizational resistance to change[10]. As companies increasingly adopt these technologies, the focus remains on refining models to enhance scalability and adaptability. Looking ahead, advancements in Internet of Things (IoT) integration and sustainability efforts promise to further revolutionize supply chain and logistics management through predictive analytics, paving the way for more efficient, responsive, and environmentally-conscious operations[11].
Predictive Models in Supply Chain
Predictive models have become essential tools in enhancing the efficiency and reliability of supply chains. These models leverage advanced algorithms and data analytics to anticipate demand fluctuations, optimize inventory levels, and identify potential disruptions, thereby ensuring a stable and reliable supply chain with minimal disruptions and high customer satisfaction[1][2]. In logistics and transportation management, predictive analytics are prominently used to aid supply chain managers in making proactive decisions and promptly responding to changing market conditions[1].
Techniques and Algorithms
Various predictive modeling algorithms, such as logistic regression, time series analysis, and decision trees, are employed to analyze data and forecast outcomes. These models can be categorized into unsupervised and supervised models, with each offering distinct methodologies for classifying data and predicting trends[3]. Machine learning techniques further enhance demand forecasting by utilizing historical data, market trends, and external variables, thereby mitigating issues like understocking and overstocking[4][5].
Applications in Inventory and Logistics Management
Predictive models are instrumental in inventory management, where they forecast demand for specific products and determine optimal restocking times, thus minimizing carrying costs and avoiding stockouts[5]. They also optimize delivery routes in real-time, accounting for variables such as traffic and weather, which results in reduced fuel costs and delivery times[4]. By integrating model-generated forecasts into logistics procedures, companies can plan deliveries, adjust stock levels, and optimize routes more efficiently, achieving observable advantages such as cost savings and improved operational effectiveness[6].
Business Impact and Challenges
The integration of predictive models in supply chains can significantly increase delivery accuracy and enhance logistics efficiency, leading to revenue generation and competitive advantage. For example, models developed using historical and multicarrier shipment data have achieved high predictive accuracy and substantial revenue impact[7]. However, scaling these models poses challenges, as they need to be continuously updated to maintain accuracy and adapt to evolving market conditions[6]. By addressing these challenges, supply chain ecosystems can fully harness the benefits of predictive analytics, leading to more resilient and adaptive supply chains[7].
Machine Learning Techniques
Machine learning techniques play a pivotal role in the development of predictive models for supply chain and logistics, enhancing delivery accuracy and efficiency. These techniques involve iteratively improving the models to adapt to changing circumstances and ensure continued accuracy in predictions[8]. The use of machine learning in logistics is transformative, allowing for intelligent analysis of large datasets that enhance route planning, inventory management, and demand forecasting[4].
A critical aspect of implementing machine learning in supply chain management is the use of predictive modeling algorithms. These include logistic regression, time series analysis, and decision trees, which can be classified into supervised and unsupervised models[3]. Supervised models utilize labeled data to train models, while unsupervised models classify data based on inherent patterns, making them ideal for tasks such as clustering and association[3].
To maintain the effectiveness of these models, they must be continuously updated with new data. This involves deploying models to production environments where they can generate real-time predictions and integrating these forecasts into logistics procedures, such as delivery planning and route optimization[6]. Machine learning algorithms are crucial for these dynamic adjustments, providing the opportunity for increased automation and reduced manual intervention in supply chain operations[1].
Moreover, machine learning facilitates task automation, which reduces costs and enhances efficiency by streamlining processes and eliminating manual labor[5]. For instance, ML algorithms can optimize transport routes by analyzing real-time traffic data, thereby reducing fuel costs and ensuring timely deliveries[5].
Development and Scaling of Predictive Models
The development of predictive models in the supply chain and logistics sector begins with the collection, storage, and management of vast amounts of supply chain data, including sales, inventory, production, transportation, and customer behavior data[1]. This data forms the foundation for building models that enhance delivery accuracy and operational efficiency. The use of predictive modeling algorithms such as logistic regression, time series analysis, and decision trees is crucial for analyzing these datasets[3].
A critical step in the development process is data cleansing and preprocessing. This involves identifying and fixing inaccuracies, inconsistencies, or missing data. Techniques like normalization and smoothing are employed to remove noise and outliers, thus allowing for more precise forecasting[1]. Once the data is prepared, machine learning algorithms are utilized to improve the accuracy of the predictive models by selecting the most relevant features that reduce model complexity[1].
To ensure the models deliver observable advantages such as cost savings and better customer support, they must be regularly assessed and refined based on new data and insights[6][1]. Once the models are developed and verified, they are deployed in a production environment to generate predictions either continuously or in real-time[6]. This requires a dedicated team with specific roles, including data scientists, analysts, domain experts, and project managers, to oversee the implementation process[1].
Scaling these predictive models involves overcoming compatibility issues, data quality challenges, and organizational resistance[1]. By leveraging existing data sources and IT infrastructure, organizations can achieve a seamless integration process that enhances efficiency and avoids unnecessary investments[1]. Moreover, establishing a feedback loop and continuous improvement practices is essential for maintaining the accuracy and effectiveness of predictive analytics solutions[1].
In practice, the deployment of predictive models can lead to significant business impacts. For example, in logistics, predictive analytics can enhance delivery accuracy to 80%, resulting in increased revenue generation of approximately $1M annually. Such models are developed using historical and multicarrier shipment data, which further demonstrates the scalability and benefits of predictive delivery solutions for supply chain ecosystems[9].
Applications in Logistics
Predictive models have become an integral component of logistics, revolutionizing the way companies manage their supply chains. The adoption of predictive analytics allows logistics companies to optimize delivery routes, anticipate demand, and manage resources more effectively[2][10]. For example, UPS has integrated machine learning and artificial intelligence to enhance its package delivery operations, predicting package volumes at various stages and efficiently allocating resources, thus reducing delays and boosting customer satisfaction[4].
The implementation of predictive analytics in logistics also extends to enhancing visibility across the shipment lifecycle. Companies like Transmetrics have played a significant role in providing data-driven insights that prepare logistics operations for peak demand periods and offer precise cost management for nonstandard-sized items[9]. These analytics solutions enable the operation of 'just-in-time' supply chains, ensuring that resources are available when needed without excessive stockpiling[9].
In addition to optimizing operations, predictive models are employed for risk management, improving supplier performance, and reducing potential disruptions[1]. By using performance metrics, logistics experts can identify bottlenecks and delays, allowing for preemptive measures such as renegotiating supplier contracts or implementing contingency plans[1]. This proactive approach ensures minimal disruptions and maintains a stable, reliable supply chain, ultimately improving customer satisfaction[1].
Moreover, the development and scaling of predictive models have addressed scalability challenges within supply chain ecosystems. These models rely on historical and multicarrier shipment data to generate accurate forecasts, which can be applied across various logistics scenarios, thus driving efficiency and revenue growth[6][10]. Overall, the use of predictive analytics in logistics not only enhances delivery accuracy, achieving up to 80% predictive accuracy in some cases but also contributes significantly to revenue generation[10].
Real-World Impacts
The application of predictive models in supply chain and logistics has transformed the industry by enhancing delivery accuracy and operational efficiency. Companies implementing these models have observed significant improvements in their logistical processes, achieving delivery accuracy rates of up to 80%, which directly contributes to revenue generation, sometimes up to $1 million annually[6][10]. The integration of machine learning techniques allows for the analysis of massive datasets, offering the ability to predict customer needs and optimize supply chain operations[11].
Predictive analytics plays a crucial role in decision-making by identifying patterns and trends that can be leveraged to anticipate future outcomes, enabling companies to plan deliveries, adjust inventories, and optimize routes more effectively[6]. This approach not only ensures cost savings but also enhances customer service by providing personalized experiences and resolving common inquiries automatically[11]. Furthermore, the predictive models help logistics companies detect potential failures in machine components before they occur, thus maximizing equipment uptime and reducing maintenance costs[9].
In the context of environmental impacts, predictive analytics addresses the last-mile delivery problem, which is a major contributor to carbon emissions in logistics. By optimizing routes and predicting delivery times more accurately, companies can reduce empty truck miles and improve fuel efficiency, thus minimizing their carbon footprint[9]. The overall transparency and efficiency in the supply chain are enhanced, contributing to a more sustainable and responsive logistics ecosystem[11].
However, the implementation of these predictive models is not without challenges. Companies often face issues related to data quality, compatibility, and organizational resistance, which must be overcome to achieve seamless integration and reap the full benefits of predictive analytics[1]. Despite these hurdles, the real-world impacts of predictive models in supply chain and logistics underscore their importance as tools for achieving greater efficiency, cost-effectiveness, and customer satisfaction in a rapidly evolving market[7].
Business Impacts
Predictive models have a transformative impact on business operations within the supply chain and logistics sectors. One of the primary advantages is the ability to enhance delivery accuracy significantly. For instance, predictive delivery solutions developed using historical and multicarrier shipment data have demonstrated an 80% improvement in delivery accuracy, leading to more reliable and timely deliveries[8]. This level of accuracy is crucial for businesses aiming to meet customer expectations and maintain competitive advantage in a demanding market environment[9].
Moreover, the integration of machine learning techniques with predictive analytics has proven to be a revenue-generating strategy, with some models achieving annual revenue increases of up to $1 million[8]. The predictive models enable businesses to forecast demand more accurately, optimizing inventory management and reducing costs associated with excess stock or stockouts[6]. For example, predictive analysis has the potential to cut inventory expenses by as much as 30%, thereby improving profitability and operational efficiency[6].
The fast-paced nature of modern supply chains, especially in industries like fast fashion, underscores the need for quick adaptation to changing consumer trends and preferences. Companies employing predictive models can swiftly move from concept to consumer, capitalizing on current trends and enhancing their market presence[2]. These models allow for agile responses to fluctuating market conditions, thus minimizing the risks associated with incorrect demand forecasting and inefficient resource allocation[8].
Future Trends and Developments
The future of predictive models in supply chain and logistics is poised for transformative developments driven by technological advancements and evolving market demands. One of the prominent trends is the increasing integration of predictive analytics with the Internet of Things (IoT) devices and sensors. This combination allows companies to collect real-time data on shipment conditions and locations, enhancing the precision of delivery predictions and operational efficiencies[1][12].
Machine learning continues to play a pivotal role in advancing predictive capabilities. As the machine learning market experiences significant growth, businesses are leveraging these technologies to refine data analysis processes, resulting in more accurate predictions and improved decision-making[5]. This trend is expected to strengthen as companies invest in predictive analytics, with a considerable number planning to integrate these solutions into their operations in the coming years[1].
Moreover, there is a growing emphasis on developing agile and resilient supply chains to effectively respond to unforeseen disruptions, such as those experienced during the COVID-19 pandemic[12]. Predictive models are central to these efforts, providing organizations with insights that allow for proactive adjustments to supply chain strategies, ultimately enhancing overall efficiency and transparency[10].
The integration of predictive models also aligns with the broader push towards sustainability in logistics and supply chain management. By optimizing routes and reducing delays, companies can minimize their carbon footprint, contributing to environmental sustainability while improving delivery accuracy[12].
Furthermore, scalability remains a key focus area, with businesses seeking to extend the benefits of predictive models across diverse supply chain ecosystems. This involves overcoming challenges related to data integration and model adaptation to accommodate different operational contexts, ensuring that the advantages of predictive analytics are maximized at every level of the supply chain[7][10].
References
[1] Raj, A. (2023, December 6). Supply chain predictive analytics: Benefits, use cases and growth potentials. ThroughPut AI. https://throughput.world/blog/predictive-analytics-in-supply-chain/
[2] Hayes, A. (2023, July 25). The supply chain: From raw materials to order fulfillment. Investopedia. https://www.investopedia.com/terms/s/supplychain.asp
[3] Lawton, G., Carew, J. M., & Burns, E. (n.d.). Predictive modeling. TechTarget. https://www.techtarget.com/searchenterpriseai/definition/predictive-modeling
[4] Acropolium. (2023, October 13). Adopting machine learning in supply chain and logistics for successful automation. Acropolium. https://acropolium.com/blog/adopting-machine-learning-in-supply-chain-and-logistics-for-successful-automation/
[5] Mukherjee, A. (2023, May 3). Machine learning techniques in supply chain management. Supply Chain Management Review. https://www.scmr.com/article/machine_learning_techniques_in_supply_chain_management
[6] Barthwal, R. (2023, December 4). Predictive analysis in logistics and supply chain: How to apply. 3PL Links. https://3pllinks.com/predictive-analysis-in-logistics-and-supply-chain/
[7] Extensiv. (2024, August 8). Supply chain challenges in 2024 & how to overcome them. Extensiv. https://www.extensiv.com/blog/supply-chain-management/challenges
[8] Aljohani, A. (2023). Predictive analytics and machine learning for real-time supply chain risk mitigation and agility. Sustainability, 15(20), Article 15088. https://doi.org/10.3390/su152015088
[9] Transmetrics. (2024, March 12). Predictive analytics in logistics: Applications & use cases. Transmetrics. https://www.transmetrics.ai/blog/predictive-analytics-in-logistics/
[10] Logistics Business. (2024, July 23). Transforming logistics with predictive analytics. Logistics Business. https://www.logisticsbusiness.com/transport-distribution/transforming-logistics-with-predictive-analytics/
[11] Shinkarenko, A. (2023, January 25). Machine learning in logistics: Top use cases & implementation. Itransition. https://www.itransition.com/machine-learning/logistics
[12] Logmore. (2019, April 2). A short history of supply chain management. Logmore. https://www.logmore.com/post/history-of-supply-chain-management
About the Author
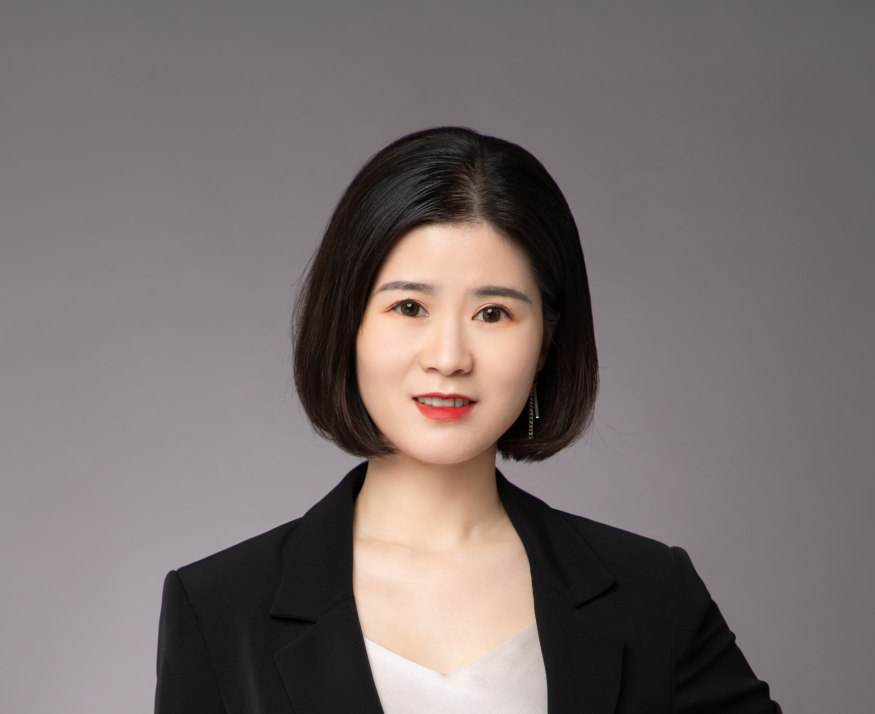
Ting Huang is a data-driven innovator and product management expert specializing in the application of predictive models and machine learning in the supply chain and logistics industry. With a strong background in leveraging advanced analytics to optimize delivery accuracy and operational efficiency, Ting's work focuses on developing scalable, customer-centric solutions that address key industry challenges. Her scholarly article, Improving Delivery Accuracy and Efficiency in Supply Chain Through Predictive Models, highlights her expertise in integrating cutting-edge technologies to transform logistics operations and drive business success. Ting holds an MBA from Washington University in St. Louis and is a recognized thought leader in digital transformation for global supply chain ecosystems.