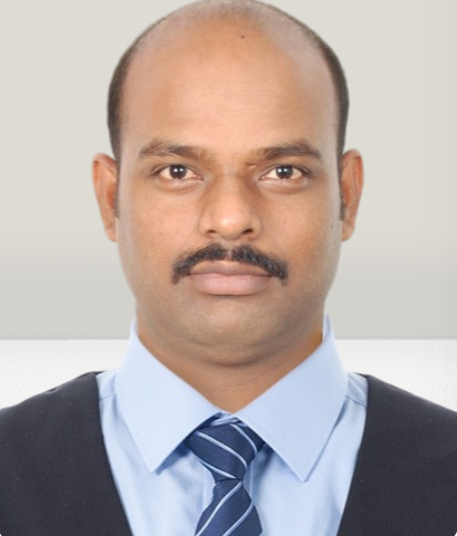
As cloud computing continues to play a stellar role in digital transformation, the importance of building strong and flexible cloud infrastructure has increased significantly. Ravi Kumar Vankayalapati, a technology expert with proven expertise in enterprise-level architecture, has made significant contributions towards addressing these challenges by leading AI-driven innovations. In one of his research papers titled "AI-Powered Self-Healing Cloud Infrastructures: A Paradigm for Autonomous Fault Recovery," he presented a new approach to artificial intelligence (AI) integration for enhancing the autonomy, efficiency, and reliability of cloud infrastructures.
Current Challenges and the Need for Automation
Cloud infrastructure serves as the nucleus of any digital service by fueling everything from enterprise software to systems that are mission-critical. However, they also pose several risk factors, such as system failures, sudden runtime faults, and service downtimes. Manual intervention is essential for addressing these issues, which can delay the recovery process and increase operational expenses. Moreover, traditional mechanisms for fault recovery are often incapable of handling modern cloud environments and their dynamic demands. This is why an autonomous and robust solution is the need of the hour.
When the cloud infrastructure is unreliable, end-users and businesses may face several issues, such as security vulnerabilities, service outages, and financial losses. With cloud ecosystems becoming increasingly complex because of hybrid and multi-cloud deployments, detection and resolution of faults can be an uphill task. In digital computing environments, another critical challenge for organizations is to ensure efficient resource management while maintaining smooth and continuous operations.
In his research paper, Vankayalapati recognizes this gap and proposes a framework for an AI-powered self-healing cloud that can autonomously detect, diagnose, and mitigate faults. This framework utilizes the capabilities of AI and ML to increase the efficiency and resilience of cloud systems by optimizing resource allocation and minimizing downtime. By relieving the IT teams from the burden of routine fault management, the framework also allows them to spend more time on important strategic initiatives.
Understanding the Concept of AI-Driven Self-Healing
The conventional strategies for cloud management rely heavily on human supervision and predetermined recovery scripts. On the other hand, self-healing infrastructures make use of AI for prediction, detection, and real-time resolution of issues.
Some key elements of this framework include
- Detection of Fault: By monitoring cloud environments continuously, AI models are able to analyze metrics, logs, and system behavior. This helps them identify irregularities before they result in system failures. As a result, service continuity can be maintained by proactive implementation of mitigation strategies.
- Diagnosis of Faults: Use of deep learning algorithms allows systems to categorize and track down the underlying causes of faults without spending much time on diagnosis. Over time, this AI-powered approach improves the accuracy of detection models by analyzing past incidents.
- Recovery: One of the greatest advantages of this framework is its ability to prioritize the most important recovery activities, such as resource allocation, automated patching, self-repair mechanisms, etc. Utilizing the techniques of reinforcement learning empowers cloud systems to adjust dynamically to new challenges. As a result, cloud infrastructures become more efficient in handling complicated faults.
Cloud in a Robot: An Evolving Concept
The research by Vankayalapati also discusses the all-new concept of "Cloud in a Robot" or "Robot Clouds" for enhancing edge computing platforms through AI-powered self-healing. Robot Clouds can reduce the latency of cloud systems and enable decision-making in real time by ensuring that data is processed closer to the source. They are also capable of dynamically optimizing computational resources, which can greatly benefit smart city infrastructures, industrial automation, autonomous vehicles, and other applications that are dependent on AI-driven analytics. Moreover, the resilience of AI-powered automation systems, medical devices, and IoT networks can also be enhanced using Robot Clouds.
Addressing Challenges
Despite the extraordinary future prospect of AI-driven self-healing cloud infrastructure, Vankayalapati has highlighted some challenges in his paper.
- Security: Robust cybersecurity measures must be implemented so that malicious actors are not able to manipulate the system.
- Scalability: It is also essential for self-healing mechanisms to be able to function efficiently across hybrid and multi-cloud infrastructures. Therefore, AI-enabled fault recovery systems must be integrated at different levels, covering distributed edge networks, data centers, and more.
- Transparency: All AI-driven recovery initiatives must look to create an environment of reliability and trust amongst all stakeholders. As AI is likely to become an integral part of cloud management, it is important for all stakeholders to understand and trust this process of decision-making.
Conclusion
Ravi Kumar Vankayalapati's insight on self-healing cloud is a huge step forward towards redefining cloud resilience. In the near future, autonomous fault recovery systems across industries can become significantly more efficient, reliable, and scalable by embracing the framework proposed by him.
"By adopting this transformative approach, technology leaders, governments, and enterprises can ensure a bright future for cloud computing. Rather than disruptions and downtime, this future will be defined by self-sustaining infrastructure and autonomous resilience," Vankayalapati mentions.
For more details, please visit this LINK.