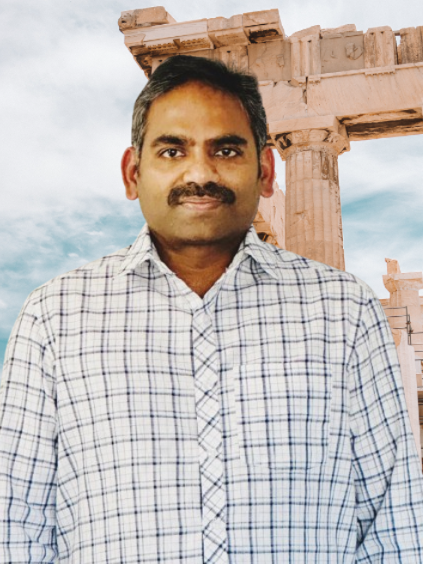
As the search for sustainable business solutions continues around the world, machine learning is steadily gaining momentum as a game changer in many different industries. Ramachandra Rao Nampalli, a noted expert in the application of AI solutions in transportation systems, strongly believes that machine learning has immense potential in transforming fleet electrification. In his recent research article, Nampalli showcased the huge potential of machine learning in fleet electrification. This article highlights how the application of machine learning models can reduce energy costs, improve fleet reliability, and enhance passenger satisfaction.
How AI Enhances Fleet Management
According to Nampalli, electrification of fleets demands much more than just using electric motors to replace internal combustion engines. It requires a complete revamp of the way our transport systems operate. With burning issues such as climate change, rapidly rising cost of energy, and ever-increasing logistical pressure, innovative solutions are essential for today's transportation systems, and this is where machine learning (ML) can make a huge difference. The introduction of ML can improve transportation systems by offering real-time optimizations and predictive insights that were unimaginable in the past.
Nampalli informs that the process of fleet electrification has its own challenges, such as ensuring the efficiency and longevity of the vehicles, balancing energy consumption, etc. Machine learning not only addresses these problems efficiently but helps build more sustainable, highly efficient, and smarter transportation systems.
"Fleet management improves the operational efficiency of the fleet, leads to sustained growth of the business, and also reduces the amount of money spent on vehicle repair and fuel consumption. Data analytics plays a crucial role in understanding the complexities involved in vehicle operations. Machine learning comprises a set of algorithms, with their roots in pattern recognition, regression analysis, classification algorithms, and unsupervised clustering," Nampalli mentions.
Applications of ML in Fleet Electrification
Through his extensive research, Nampalli has identified some critical areas of fleet management where ML can make a significant positive impact.
- Predictive Maintenance: By analyzing real-time and historical data, machine learning algorithms can forecast the probability of machine component failure. This not only reduces repair costs but also enhances safety and reduces downtime.
- Optimized Energy Consumption: With the help of AI models, charging schedules and routes can be optimized by fleets. This ensures minimum consumption of energy without any impact on the quality of service.
- Dynamic Management: Transportation systems can utilize ML-driven insights to forecast demand patterns, allowing operators to reduce congestion by efficient resource allocation during peak hours.
- Battery Health: Maintaining proper battery health is a critical requirement for all-electric vehicles. ML models are extremely effective in battery performance monitoring, degradation forecasting, and extending battery life by suggesting optimal patterns for charging.
- Driver Feedback: It is possible to provide real-time feedback to drivers about their driving behavior by using AI-enabled tools. This feedback can help reduce vehicle wear and tear and encourage energy conservation practices.
Fleet Electrification Case Studies
In his article, Nampalli presented some case studies that involved the application of machine learning for electrification and management of fleet. These case studies covered many different industries, such as vehicle rentals, refuse collection, and public transportation. In all these projects, machine learning was applied successfully by the stakeholders to reduce fuel consumption, optimize routes, monitor the use of energy by the vehicles, and optimize the location of time of vehicle charging.
Obstacles and Future Directions
Though the potential of machine learning in fleet electrification is massive, Nampalli mentions that there can be significant implementation challenges.
- Sensitive operational data can be accessed while power demand is collected by unmanned aerial vehicles from public charging infrastructure.
- At present, machine learning models are built based on a single scenario or use cases, such as the extension of battery life, allocation of cost and revenue, and emission reduction.
- Generalization of machine learning models can be quite difficult, even when there is a minor change in fleet composition or operational environment.
- There are also ethical and technical challenges related to tracking each behavior of a fleet owner regarding their system design use cases.
"To address these challenges, researchers and practitioners should collaboratively develop few-shot learning algorithms, a unique artificial intelligence that can memorize previous knowledge with only a few examples provided. Several innovations in methodologies have advanced the applicability of machine learning in real world operational challenges. The recent breakthroughs of jointly developing machine learning and transport systems have indirectly provided us with a broad range of interesting optimization questions in novel areas, such as machine learning, supply chain, and service systems management," Nampalli states in his article.
Pioneering AI-Powered Fleet Electrification
A distinguished solution architect and AI applications specialist, Ramachandra Rao Nampalli has an excellent track record in driving innovative and sustainable transportation solutions. During his tenure with Denver RTD, his work was mostly focused on the intersection of transportation of AI. His research in the field of machine learning models and neural networks has unveiled new avenues to freight demand forecasting and optimization of passenger flow in real time.
Nampalli's vision extends way beyond the immediate advantages of fleet electrification. His goal is to help build futuristic transport systems that are not just efficient but also highly scalable and can adapt to future demands.
FOR MORE DETAILS, VISIT THIS LINK.