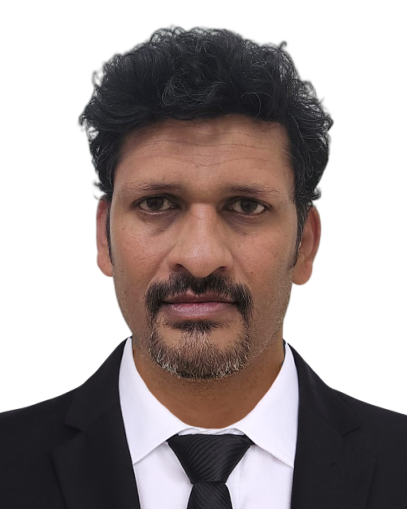
In today's rapidly evolving financial landscape, credit card fraud is one of the most pervasive crimes that cost billions of dollars every year to financial institutions and businesses across the globe. As more and more people embrace digital payments, fraudsters have upped their game to exploit the vulnerabilities in mobile banking, online transactions, and e-commerce platforms.
A recent study by Lakshminarayana Reddy, an industry leader currently working as Assistant Vice President at US Bank, explores the behavioral trends in the context of credit card fraud. It also examines the potential of privacy-preserving AI and federated learning in mitigating this crisis.
Limitations of Traditional Fraud Detection
Recent reports suggest that the losses suffered each year because of worldwide incidents of payment fraud may exceed $40 billion by the year 2027. As technology has progressed over time, fraudsters are now proficient in evading detection strategies by deploying deepfake identity fraud, AI-driven attacks, and synthetic identity schemes. It is impossible to fight these threats by employing traditional systems relying on static algorithms and rule-based mechanisms.
Reddy's research paper explains why conventional fraud detection often fails to deliver the desired results.
- Reactive in nature, rule-based systems operate based on predefined rules, which can be easily bypassed by copying legitimate behavior.
- With rapidly changing patterns of fraud, new types of fraudulent behaviors can't be detected by models without continuous learning capabilities.
- In some instances, even legitimate transactions may get flagged by detection systems as fraudulent, which leads to poor customer experiences.
- Financial institutions are often afraid of privacy violations and regulatory penalties. As a result, they are reluctant to share any information related to fraud.
Changing the Game with AI
The good news is that the finance industry can now conduct real-time fraud detection, analyze behavioral patterns, and deal with emerging threats using tools powered by AI and ML. Capable of processing millions of transactions per second, AI models are extremely proficient in identifying anomalies, hidden fraud networks, and suspicious spending behavior with exemplary efficiency.
The fraud detection model proposed in Reddy's paper combines AI with privacy-preserving AI and federated learning, which helps counter financial fraud while preserving consumer data.
Understanding the Role of Federated Learning
Federated learning is one of the important innovations in the field of AI that represents a new approach to machine learning. Focused on privacy, this approach is all about encouraging collaboration in the detection of fraud without sharing raw data related to transactions.
According to Reddy, unlike traditional models relying on centralized datasets, decentralized model training is enabled by federated learning. This fraud detection methodology is discussed below.
- The AI model of each bank gets trained locally using the bank's own transaction data.
- Banks only transmit the updated model parameters, such as insights and patterns, to a central system.
- The updates received from different institutions are aggregated by AI models. Therefore, even without accessing any sensitive data, the models can learn from diverse patterns of fraud.
- Finally, the enhanced model is shared with all participating banks.
"Federated learning brings a paradigm shift in fraud detection by allowing institutions to collaboratively fight cybercrime without jeopardizing consumer privacy," said Reddy. "This approach is critical to the development of high-accuracy fraud detection models while avoiding the risks related to centralized storage of data."
Securing Transactions with Privacy-Preserving AI
Though there have been significant advances in AI-driven systems for fraud detection, the privacy of consumers continues to be the foremost concern. In his paper, Reddy has discussed different techniques of privacy-preserving AI for detecting fraud while shielding sensitive information.
- Homomorphic Encryption: With this encryption technique, it is possible to detect fraud while maintaining the confidentiality of data through AI models capable of analyzing encrypted data without any need to decrypt them.
- Differential Privacy: It allows AI models to accurately detect fraudulent activities without linking these transactions to any particular individual.
- Secure Multi-Party Computation (SMPC): This technique allows multiple financial institutions to collectively compute patterns of fraud without revealing the transactions of any individual consumer.
Benefits for Financial Institutions
The findings of this research are likely to have remarkable implications for banks and financial institutions in terms of fraud prevention and privacy compliance.
- By preventing transactions from taking place, AI-powered fraud detection helps avoid chargebacks and financial losses.
- Improves customer satisfaction by ensuring that legitimate transactions are not flagged or restricted.
- Without exposing any sensitive information, federated learning fosters close collaboration between institutions for preventing fraud.
- By evolving continuously, AI models can help outsmart cybercriminals by detecting the latest and emerging patterns of crime.
"These are exciting times for the finance sector as it gets closer to a turning point," Reddy asserts. "With the advent of AI-enabled fraud detection, financial institutions can now protect customers, ensure data privacy, and reduce financial losses without compromising efficiency or security."
Future Opportunities
The research byLakshminarayana Reddy identifies several future opportunities for advancing the impact of AI on financial security.
- Enhancing fraud detection by combining transaction data, geolocation analysis, and behavioral biometrics.
- Expansion of AI models for the detection of blockchain-related scams and fraudulent crypto transactions.
- Creating a global network of financial institutions for fraud prevention.
- Making fraud detection decisions more transparent through explainable AI techniques.
For more details about Lakshminarayana, please visit the Link.