A recent study paper released by Fitbit indicates that the device may be used to diagnose COVID-19 by detecting the wearer's biometrics sooner than possible from self-reported signs.
Researchers studied patterns in heart rate, step count, and symptom length between patients with flu and those with COVID-19 by reviewing Fitbit data and self-reported symptoms.
It is essential for COVID-19 screening to contrast and associate to influenza and COVID-19, as existing procedures mostly search for more general symptoms such as fever.
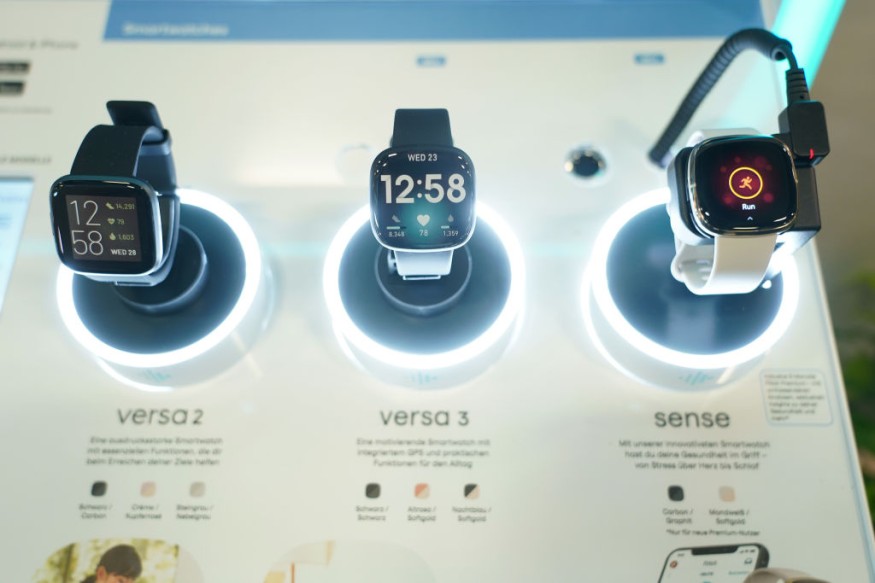
There are too many factors that could cause COVID-19: Expert
Senior author Luca Foschini, co-founder of United States-based Evidation Wellness, noted that certain screening checks at building entrances are all temperature-based. He said several people don't instantly catch a fever because there are too many factors other than COVID-19 that induce fever.
A major increase in the resting heart rate is a more sensitive measure of COVID-19, according to Foschini. He indicated that individuals with activity trackers might exchange that data for monitoring purposes with approval, much like having a temperature reading.
Foschini's study suggested that many other signs, such as shortness of breath and coughing, are typical of COVID but not influenza. They also analyzed each disease's influence on the decrease of the regular phase count, noting that for COVID, the results continued much longer than for flu.
Although data from wearables like Fitbit can show a lot about these respiratory diseases, the researchers suggest that it should be used not as a full diagnosis tool, rather as a general screening process.
How does Fitbit exactly detect the difference between COVID-19 and flu?
Experts whose study is on npj Digital Medicine describes the early signs of disease observed by respiratory rate, pulse rate, and variability of heart rate measures (HRV). The researchers found that around 11 and 12 percent of 2745 participants who had positive for COVID-19 with PCR swab tests were asymptomatic. A total of 21 symptoms were reported, while just over half registered fevers.
The researchers noted an expected hospitalization based on self-reported symptoms with an AUC of 0.82 or a probability of ±0.017. The disease was predicted with a precision of 0.77 ±0.018 AUC on a particular day based on physiological results.
They usually find that although HRV is reduced, respiration and heart rate are increased by the disease. To use these metrics as predictive variables, a neural network was educated.
The researchers conclude that calculating these metrics can contribute to better early COVID-19 detection and monitoring, along with molecular-based diagnosis and treatment.
The study paper goes through some depth regarding the possibility of various signs and the length of different intensity cases. Stanford University and Scripps Translational Science Center have also reportedly been collaborating with Garmin for further studies.
ALSO READ : First Time in the World: Demonstration of Ultra Pressure Sensor Attached to the Fingertips
Check out more news and information on COVID-19 and Technology on Science Times.