Artificial intelligence (AI) models in the past decades have used deep learning algorithms for a variety of applications. Now, a new AI tool could help analyze pharmaceutical drugs and predict their future properties.
A team of researchers from Tsinghua University in Beijing, Fudan University's School of Pharmacy in Shanghai, and Zhejiang University in Hangzhou - all in China, and the National University of Singapore have developed MolMapNet - a new AI tool that uses out of the box deep learning to predict the pharmaceutical properties of various drugs by analyzing molecular representations collected by human knowledge bases.
Details of MolMapNet are reported in the article "Out-of-the-box deep learning prediction of pharmaceutical properties by broadly learned knowledge-based molecular representations," now on the journal Nature Machine Intelligence.
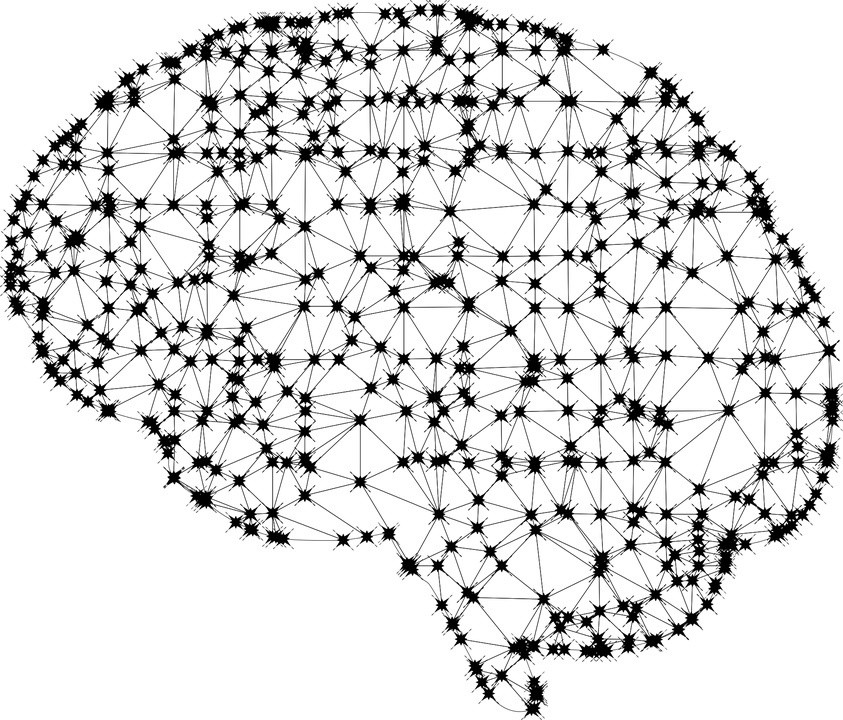
Studying the Vast Collection of Molecular Properties
The challenge with teaching MolMapNet is that the molecular properties are generally a set of unordered data. AI tools over the years have been designed to pour through sets of organized datasets, or using machine vision to recognize and "learn" images that are spatially ordered, where target items are arranged or are analyzed following a predetermined pattern.
"We were aware that pharmaceutical investigations require the learning of many molecular characters, particularly the rich collection of molecular properties (like volume) derived from human knowledge, but these molecular properties are tough to learn by AI (artificial intelligence)," said Yu Zong Chen, one of the authors of the study from the National University of Singapore, in an interview with TechXplore.
To surpass this challenge, researchers examined a variety of possible solutions, all with the intent of improving future deep-learning models for learning unorganized data or predicting pharmaceutical properties in the case of MolMapNet.
"With limited pharmaceutical data, it is hard to improve AI architectures," Chen added, sharing that the researchers inquiring whether they could do something to improve how AI recognizes molecular properties. They came up with mapping unordered molecular properties into more ordered images, therefore making them easier for AI tools to recognize.
Out-of-the-Box Innovation
Researchers arrived at an out-of-the-box deep learning solution that does not require fine-tuning the AI parameters, which means that the solution does not require specialized expertise or knowledge about AI programming. Furthermore, researchers discovered that their solutions surpassed other state-of-the-art AI tools in terms of recognizing most of the 26 pharmaceutical benchmark datasets.
Chen explains that their approach for MolMapNet uses three steps in arriving at the out-of-the-box deep learning improvement. The first is broadly learning the "intrinsic relationships of molecular properties from over 8 million molecules," explaining that there might be a connection between these "relationships" and thus help narrow down potential pharmaceutical properties.
Next is the use of a newly-developed data transformation technique. With this, researchers were able to map the molecular properties of different pharmaceuticals into 2D images, with pixel layouts reflecting intrinsic relationships between these different properties.
The last step is in training a machine vision tool to "learn" the mapped images, with the resulting AI system using the data to predict pharmaceutical properties. The AI tool has been designed to recognize pixel layout patterns to determine pharmaceutical properties.
RELATED ARTICLE : Naturally Chiral Surfaces Could Lead to Safer Pharmaceuticals
Check out more news and information on Pharmaceuticals in Science Times.