A new AI tool uses a combination of machine learning, satellite imagery, and weather data to autonomously find locations with heavy air pollution down to the exact city block.
Researchers at Duke University developed the AI tool, which could help researchers and respondents identify and preemptively mitigate sources of dangerous aerosols, conduct a more targeted study on air pollution effects on human health and environment, and making more informed and socially just public policies.
The Duke University team present their findings in the article "Local PM2.5 Hotspot Detector at 300 m Resolution: A Random Forest-Convolutional Neural Network Joint Model Jointly Trained on Satellite Images and Meteorology," appearing in the journal Remote Sensing.
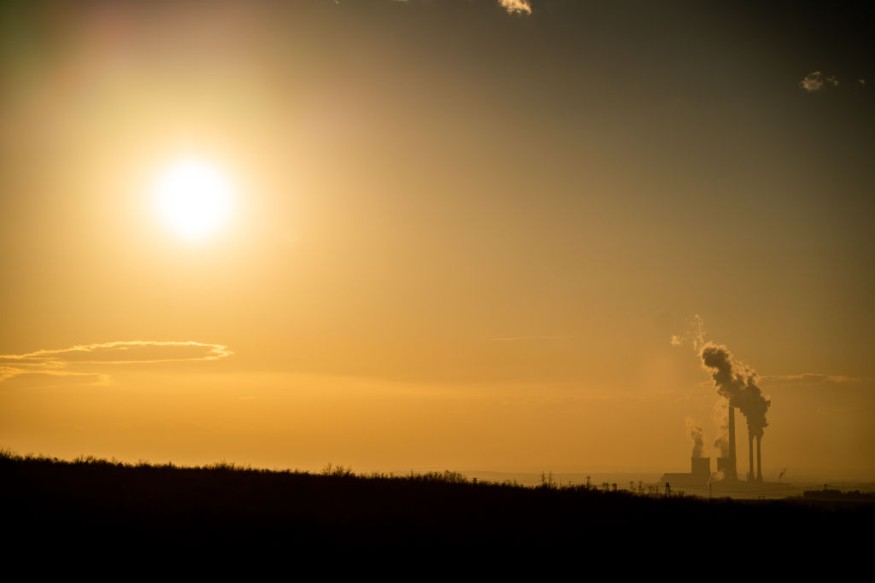
Problem With Harmful Particulate Matter in Air
"Before now, researchers trying to measure the distribution of air pollutants throughout a city would either try to use the limited number of existing monitors or drive sensors around a city in vehicles," said Mike Bergin, one of the authors of the study and a professor of civil and environmental engineering at Duke, in an article from the university. Noting that conventional methods that include sensor network setups are "time-consuming and costly," Bergin explains that the use of satellite imagery in finding air pollution hotspots is an advantage.
Particularly, researchers behind the new AI tool are interested in detecting levels of particles called PM2.5. These are fine, inhalable particles generally less than 2.5 micrometers (um) in diameter - only three percent of the diameter of a strand of human hair. Furthermore, PM2.5 particles can travel deep into the lungs once inhaled.
A report from the Global Burden of Diseases places PM2.5 in the fifth place for mortality risk factors back in 2015. It also noted that the particulate substance caused about 4.2 million deaths in a year, and another 103.1 million years of human life lost or lived in disability. A 2020 update on the study reports that more than 90 percent of the global population currently lives in locations that exceed the air quality guidelines pertaining to these minute particles.
Also, Harvard University T.H. Chan School of Public Health released a 2020 study showing that areas with higher PM2.5 levels are linked to higher death rates from COVID-19.
Weather Data, Satellite Imagery, and Machine Learning for Air Pollution Tracking
Bergin also points out a problem: that most cities are not equipped with enough ground stations if at all, owing to the facilities' costs.
While these facilities can give a general idea of air pollution conditions in a location, they are not capable of providing a "true distribution" for residents living in different parts of the same city.
Focusing on the city of Beijing, China, researchers created a measurement tool that utilizes satellite imagery and weather data in a machine learning program to measure PM2.5 measurements on smaller, more targeted locations - in the range of 300 meters, or about the size of a city block.
The automated air pollution tracker employs a method called "residual learning," starting with "learning" the PM2.5 levels from weather data alone. Next, it compares the weather data estimates with actual PM2.5 readings, fine-tuning its capabilities to read satellite images and make more accurate predictions.
RELATED ARTICLE : Air Pollution Can Affect Your Cognitive Skills Up to 60 Years Later
Check out more news and information on Air Pollution in Science Times.