Current space explorations use artificial intelligence (AI) and machine learning in many areas of space operations, such as planning of missions, data collection, autonomous navigation and maneuvering, and spacecraft maintenance. It is most likely that future space missions will need to rely on this technology.
Researchers from King Abdullah University of Science & Technology (KAUST) and The Chinese University of Hong Kong have developed a Moon-scanning technology that uses machine learning and AI to scan the lunar surface and identify the optimal site for landing and exploration.
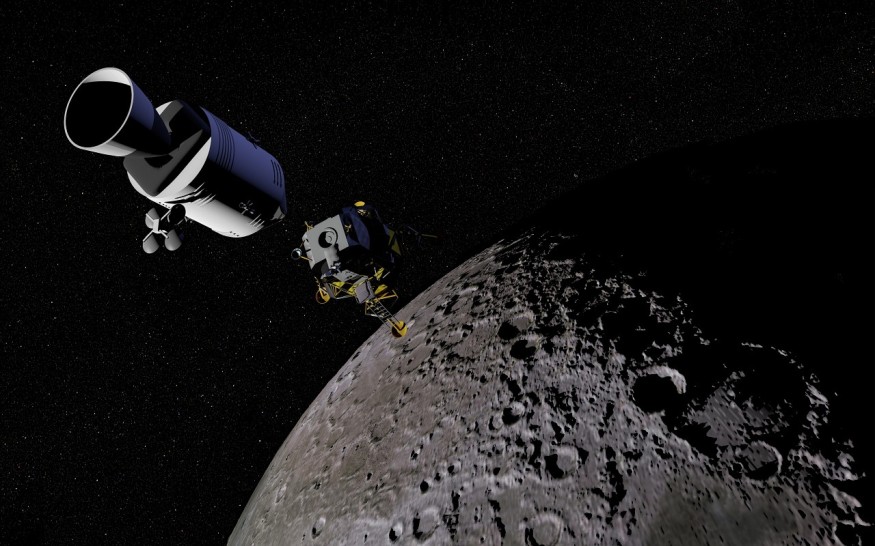
Choosing Lunar Landing Sites
How did NASA choose lunar landing sites? Why does it matter to choose the best landing site?
Before the Apollo missions, scientists only have pixelated pictures from probes that do not necessarily help space engineers to spot the best landing sites. The Moon has craters deeper than the Mariana Trench and mountains that are taller than Mount Everest, so choosing the optimal landing site for a three-legged Lunar Module will not be easy.
According to the website Hack the Moon, Moon landing sites had to be carefully identified based on the area's topography, and the trajectory and flight path of the Lunar Module. Even the time of the launch is carefully calculated as the shadow cast by the Sun could hide important details for the landing.
Choosing different landing sites will offer flexibility in takeoff, and crews will no longer have a hard time preparing for a single site when they could launch at a few different sites if ever some delays on Earth make one site inaccessible.
But scanning the lunar surface for the optimal landing site is laborious and often inaccurate. That is why scientists have applied machine learning and AI to automate the identification of prospective lunar landing and exploration sites.
Using AI and Machine Learning for Finding Lunar Landing and Exploration Sites
Siyuan Chen, one of the scientists involved in the study, said they are looking for lunar features like craters and rilles that are hotspots for uranium and helium-3, promising resources for nuclear fission. On the other hand, they learned that lunar craters are also valuable resources to replenish spacecraft fuel.
They believe machine learning could be an effective technique for training the AI model to look for these lunar features, EurekAlert! reported. However, there is no labeled dataset for the rilles that could be fed into their AI model.
So the team constructed their own training dataset with annotations for both craters and rilles using the transfer learning approach to train the AI. Their new method will not need any human intervention, unlike previous approaches, and also allow them to construct a large-high-quality dataset.
The next problem they are working on is identifying craters and rilles at the same time, something that was not done before. Chen said that they solved this problem by developing a deep learning framework dubbed as the high-resolution-moon-net. It is composed of two independent networks that share the same network architecture to identify lunar features simultaneously.
According to the news outlet, the team's method has precision as high as 83.7%, which is higher than the state-of-the-art methods used in detecting lunar craters.
The team published their study, titled "Lunar features detection for energy discovery via deep learning" in Applied Energy.
Check out more news and information on Artificial Intelligence in Science Times.