Mathematics is present throughout the various aspects of the physical world, whether we like it or not. In every corner of the planet. The language of mathematics can be found in simple sound waves, environmental features, and even in developing zebrafish embryos.
Cornell University's Center for Applied Mathematics expert Alex Townsend explained that Isaac Newton's calculus principles have allowed the modern world to derive other calculus equations known as differential equations that help us understand more about physical phenomena.
Partial Differential Calculus and Deep Learning
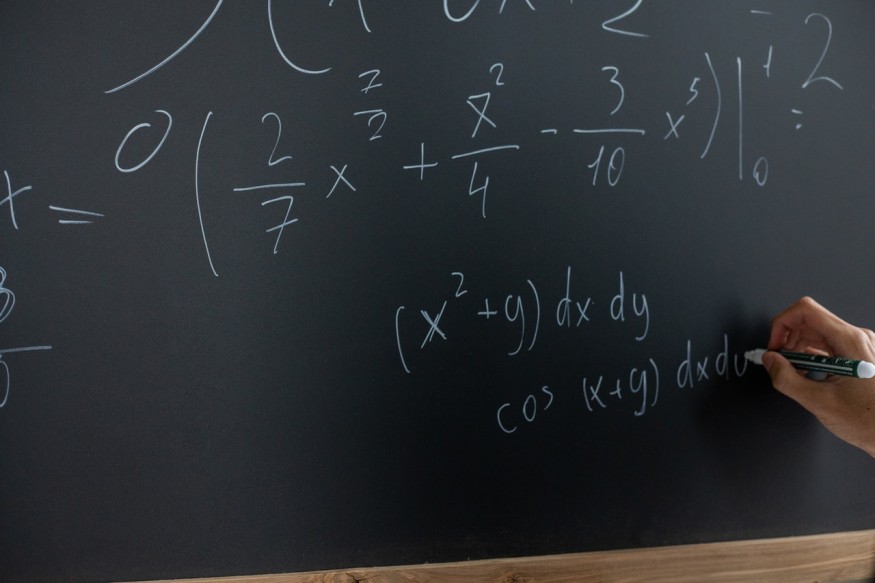
By utilizing the laws of how calculus works, Townsend said that the concept of a system's physics is already present. However, some physical systems remain unsolved.
Through the years of mathematical studies, the developing field of partial differential equation learning, or PDE, allowed mathematicians to procure information offered by the natural systems. From here, experts use machine learning technology through trained computer neural networks, which can present new mathematical equations.
In a new study, Townsend, along with collaborators from Cornell and the University of Oxford, has come up with a model of a new neural network that functions with rationality. The main goal of this innovation is to relay data to mathematics specialists in a more understandable language, all through the principles of a right inverse in differential calculus, Science Daily reports.
The association between machine learning capabilities and human knowledge is believed to be a stepping stone to deep learning, allowing mathematical studies to explore major natural phenomena in everyday life. Experts target climate change, genetics, weather systems, fluid dynamics, and many other fields from which equations would be derived.
The underlying dataset in machine learning is composed of neural networks that function similarly to the brain mechanisms in animal species. Townsend explained that these functions were heavily inspired by animal neurons and synapses, making a simple process of inputs and outputs.
In the model, the neurons serve as 'activation functions' that harvest information from other neurons. Like in the brain, neurons connect through synapses, which in the neural networks are called weights.
Neural Network Links Machine and Human Knowledge
Through the simple connection between the activation functions and weights, Townsend explained that a more detailed and complex map could materialize, having the capacity to process multiple inputs and outputs similar to how our brain works when the eyes perceive a subject and turn it into an idea.
Townsend's team's model allows us to watch a system, particularly the PDE, as it carries out estimates based on Green's function patterns and eventually predicts future concepts.
The mathematics community has studied Green's functions for over two centuries now. Today, the peak utilization of this system is to provide a solution for a differential equation rapidly. Many studies also suggested utilizing Green's functions to explain a differential equation instead of solving it.
Townsend explained that for each system, a distinct physics is present. This allows machine learning to understand Green's functions to define natural systems around us. The study was published in the journal Scientific Reports, titled "Data-driven discovery of Green's functions with human-understandable deep learning."
RELATED ARTICLE : Artificial Intelligence System by French Startup NukkAI Defeats Eight World Champions of Bridge Card Game
Check out more news and information on Artificial Intelligence in Science Times.