A new study offers a more precise approach to calculating carbon dioxide emissions, comparing factors that impact them, and testing two approaches for reducing them.
As specified in an IEEE Spectrum report, machine-learning models are exponentially growing larger. At the same time, they necessitate exponentially more energy to train so they can precisely process texts, images, or videos.
RT from IEEESpectrum: "Researchers are starting to quantify and compare the #carbonfootprint of #machinelearning training models, the first step in controlling emissions from energy-intensive #AI computing. https://t.co/rtP7lIjOgj"
— IEEE YP Central TX (@ieeeypctx) June 26, 2022
As the artificial intelligence community wrestles with its environmental effect, some conferences are asking researchers to include information on CO2 emissions.
Many software packages calculate the carbon emissions of AI workloads. Recently, a Université Paris-Saclay research team tested a group of these tools to determine if they were dependable. As a result, according to Anne-Laure Ligozat, co-author of that study who was not part of this new work, "they are not reliable at all."
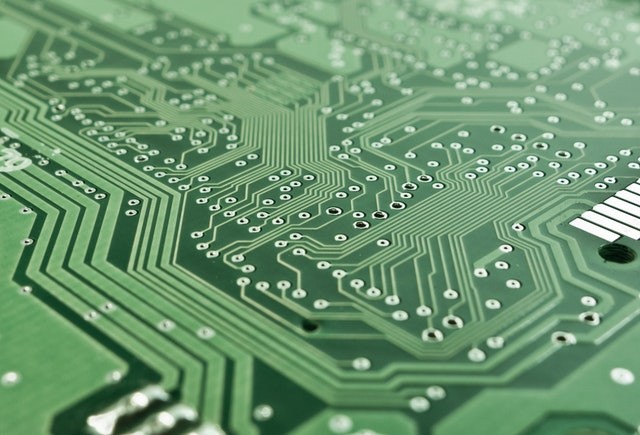
Measuring Energy Consumed
The new method, research scientist Jesse Dodge from the Allen Institute for AI and the lead author of the new paper presented recently at the ACM Conference on Fairness, Accountability, and Transparency or FAccT said, "differs in two respects.
First, it records the energy usage of the server chips as a series of measurements instead of summing their use over the period of training.
Second, it aligns such usage data with a series f data points specifying the local emissions per kilowatt-hour of energy consumed. This number changes continually, as well. Dodge elaborated that previous work does not capture many of the nuances there.
In addition, the new tool is more sophisticated than the older ones, although it still tracks some of the energy consumed in training models. In a preliminary study, the research team discovered that the GPUs of a server used 74 percent of its energy.
GPU Usage
Essentially, CPUs and memory utilized a minority, and they back many workloads simultaneously, so the researchers focused on GPU usage.
They did not gauge the energy used to construct the computing equipment or to cool the data center or develop it and transport engineers from and to the facility.
Powering the GPUs to train the tiniest models released roughly as much carbon as charging a device. Meanwhile, the biggest model had six billion parameters, a measure of its size. While training it just to a 13-percent completion, GPUs emitted nearly as much carbon to power an entire home for one year.
On the other hand, some deployed models, like the GPT-3 of OpenAIs, have over 100 billion parameters. In a similar World-Energy report, it was specified that the biggest factor in reducing emissions was a geographical region, specifically, grams of CO2 per kWh ranging from 200 to 755.
CO2 Metric Executed
Microsoft, whose researchers teamed on the paper, has already executed the CO2 metric into its Azure cloud service.
With the given information, users might decide to train at different times or areas, purchase carbon offsets, train a different model, or no model.
According to Dodge, he hopes that the vital initial step toward a greener and more equitable future is "transparent reporting," since one cannot improve what cannot be quantified or measured.
Related information about AI used in computing carbon footprint is shown in Anuj Shah's YouTube video below:
RELATED ARTICLE : NVIDIA Omniverse and the Important Role It Plays in All Metaverses
Check out more news and information on Artificial Intelligence in Science Times.