Microscopic materials characterization is crucial to achieving desired performance in next-generation nanoelectronic devices, such as low power consumption and fast speeds. However, such devices' magnetic materials frequently show intricate interactions between magnetic domains and nanostructures. Functional design is hence demanding.
Historically, scientists have examined tiny picture data visually. However, this frequently results in a qualitative and highly individualized interpretation of such data. A causal explanation of the mechanisms behind the intricate interactions in nanoscale magnetic materials needs to be included.
In a recent development that researchers reported in Scientific Reports, a group of researchers from Tokyo University of Science in Japan under Prof. Masato Kotsugi was able to automate the analysis of the microscopic picture data.
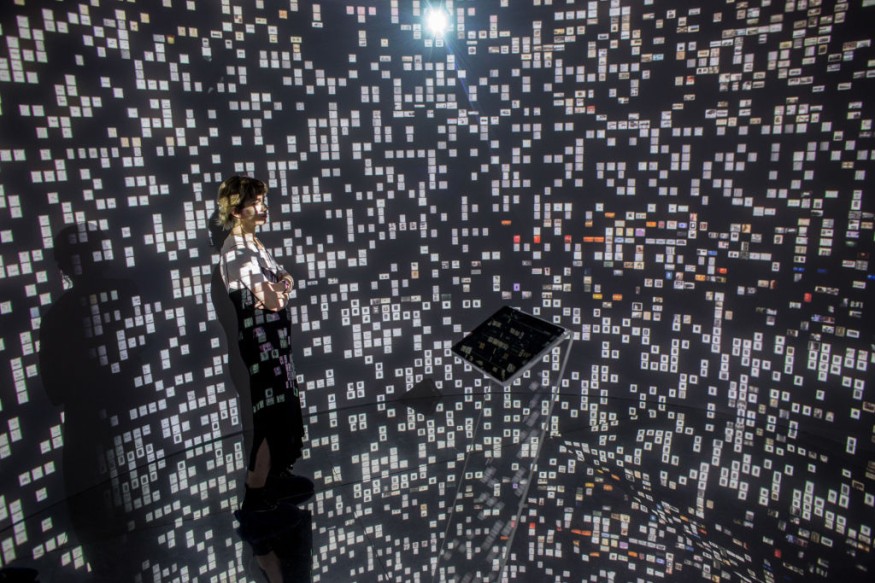
Landau Free Energy Model
The researchers used a mix of topology, data science, and free energy to create an "extended Landau free energy model," which was used to achieve this. The model suggested the ideal form for a nanodevice and could demonstrate the magnetic effect's physical mechanism and critical position.
The model drew energy landscapes in the information space using physics-based characteristics, which experts may utilize to comprehend the intricate interactions occurring at the nanoscale in various materials.
"Conventional analysis are based on a visual inspection of microscope images, and the relationships with the material function are expressed only qualitatively, which is a major bottleneck for material design," Kotsugi explained per Phys.org.
"Our extended Landau free energy model enables us to identify the physical origin and location of the complex phenomena within these materials," Kotsugi added.
Kotsugi further added that this method circumvents the explainability issue that deep learning encounters, which is analogous to generating new physical laws.
The researchers extended the Landau free energy model using state-of-the-art techniques from topology and data science domains. The result was a model that made it possible to determine the cause of the magnetization reversal in nanomagnets. The group automatically identified the physical origin and saw the initial magnetic domain pictures.
Magnetic Effect
Their findings suggested that the "pinning phenomenon" is caused by a magnetic effect created by the demagnetization energy close to a flaw. In addition, the team accomplished a feat that had not been done before: they could see where energy barriers were concentrated spatially.
According to Mirage News, the group also suggested designing recording devices and nanostructures with minimal power consumption in a topologically inverse way.
The study's model is anticipated to be useful for various applications, including spintronic devices, quantum information technology, and Web 3.
Check out more news and information on Artificial Intelligence in Science Times.