With the advancement of science and engineering, much focus has been recently given to nanosystems as it is considered one of the developments in modern science that can revolutionize most industrial processes. However, some challenges must be considered, such as system operation energy efficiency.
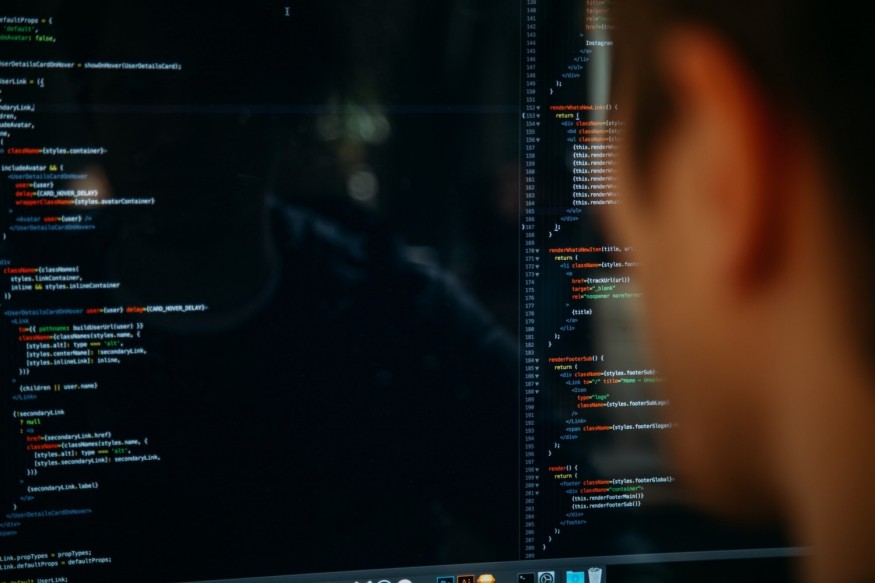
Ensuring Energy Efficiency Through Machine Learning
In a study published by Berkeley Lab, researcher Stephen Whitelam from Lawrence Berkeley National Laboratory of the Department of Energy proved that developing an improved energy efficiency for complex systems such as data centers is now possible. Whitelam used neural networks in computer simulations to train nanosystems to work with greater energy efficiency. The research was conducted at the Molecular Foundry, the DOE Office of Science user facility at Berkeley Lab.
The simulations reveal that learned protocols can draw heat from the systems by constant measurement to identify the most efficient operations. This discovery applies to operating large systems such as computer data centers. Enormous amounts of heat produced by banks of computers are extracted to avoid damaging the sensitive electronics.
Whitelam got his idea from the retro arcade game Pac-Man, which seems suited naturally to materials science. In this video game, machine learning aims to select a particular time for an action to be performed. After some time, the machine learning algorithms will eventually learn the best moves as well as the right time of execution to achieve high scores. Whitelam believes that the same learning algorithms can be applied to nanoscale systems.
The simulations developed by Whitelam also seem to answer the thought experiment in physics with a hypothetical intelligent being called Maxwell's Demon. Created by mathematician James Clerk Maxwell to contradict the second law of thermodynamics, Maxwell's Demon baffles scientists as they try to address the idea of extracting work or energy from seemingly nothing.
Using his simulation, Whitelam has proven that Maxwell's Demon does not violate the second law of thermodynamics because the information is equivalent to energy. If the machine learning protocol is considered the demon, it can convert information taken from the system modeled into energy as heat.
Whitelam also applied his idea to microelectronics and computing. According to him, the simulations he developed are similar to a testbed to enable the understanding of concepts and ideas. He believes his idea only shows that it is possible to perform these protocols using measurements that can be applied in real-life investigations.
READ ALSO : Researchers Develop Machine Learning Algorithm to Help Further Understand Quantum Systems
Achieving Energy Efficiency in Nanoelectronics
Microscale and nanoscale technology enable transformative networks for self-powered systems. Despite the proven benefits of this breakthrough, its success still depends on delivering adequate power and energy to autonomous systems. According to the Taylor & Francis Group, the future of nanoelectronics must be conscious of energy efficiency as it will significantly affect the global energy-saving balance.
RELATED ARTICLE : Standards in Machine Learning Key to Continuous Advancement, Requires Trust Among Researchers
Check out more news and information on Machine Learning in Science Times.