Artificial intelligence can perform digital calculations by using neural networks with the help of microelectronic chips. In a recent study, researchers created a neural network that uses active colloidal particles instead of electricity.
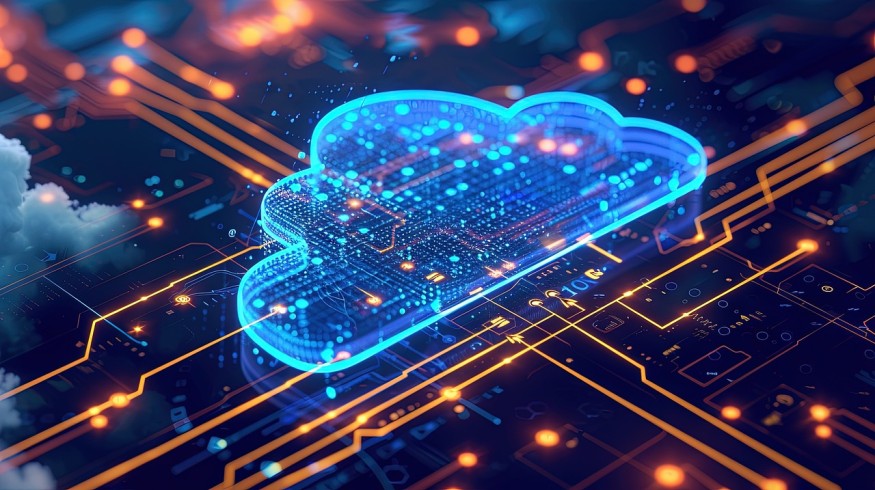
Information Processing With Active Networks
Processing and storing of information is a significant aspect of any living system. They are involved in many biological processes, such as computations in large biological neural networks in the brain. These feedback mechanisms control and maintain the functions of organisms and the detection of low amounts of chemicals needed by bacteria to navigate the environment.
With living matter as the substrate of computation, all these processes depend on its inherent activity. They inspired computational models of artificial intelligence executed on well-designed electronic hardware that uses completely different representations of information.
Recurrent neural networks are one variant of these mathematical algorithms with fading memory that enables learning from information sequences. Sparsely and statistically connected recurrent nodes are used by reservoir computers to create a high-dimensional space.
While recurrent nodes feature a memory of past states, reservoir computers enable physical realization on uncommon computational substrates. This inspires experts to explore microscopic reservoir computing in synthetic active microsystems using active living systems.
READ ALSO : Adversarial Neural Network Training Aids in Understanding How Powerful Artificial Intelligence May Work
Utilizing Active Microparticles
At Leipzig University, physicists described how microparticles can be utilized as a physical system for AI and time series prediction. Their findings are reported in the paper "Harnessing synthetic active particles for physical reservoir computing."
According to Professor Frank Cichos, their neural network makes calculations using the dynamics of physical processes. They used synthetic self-propelled particles, which measure only a few micrometers.
In this study, the research team demonstrated that the microparticles can be used for calculations while presenting a method that suppresses the impact of disruptive effects in the motion of colloidal particles. For the experiment, the scientists created tiny units made of plastic and gold nanoparticles where one rotates around another using a laser. Such units possess specific physical properties, which make them interesting for reservoir computing.
While each unit processes information, a collection can make up a reservoir. To utilize their potential, the research team changed the rotational motion of the microparticles in the reservoir using an input signal. The resulting rotation contains the calculation outcome, but the system needs to be trained to perform a specific calculation.
According to Professor Cichos, the reservoir is subject to strong noise since the system contains tiny particles in water. Similar to noise that all brain molecules are subject to, this one severely disrupts the functioning of the reservoir computer and typically requires a vast reservoir as a remedy.
In their investigation, the researchers found that using past reservoir states can improve computer performance and enable smaller versions to be functional for certain computations under noisy conditions. They believe their findings have contributed to information processing with active matte and yielded a technique that can optimize reservoir computation through noise reduction.
RELATED ARTICLE : Spin-Glass Consisting of Nanomagnets Being Developed to Replicate Neural Network, a Strategy for Mathematical Thinking of Material Structure
Check out more news and information on Neural Network in Science Times.