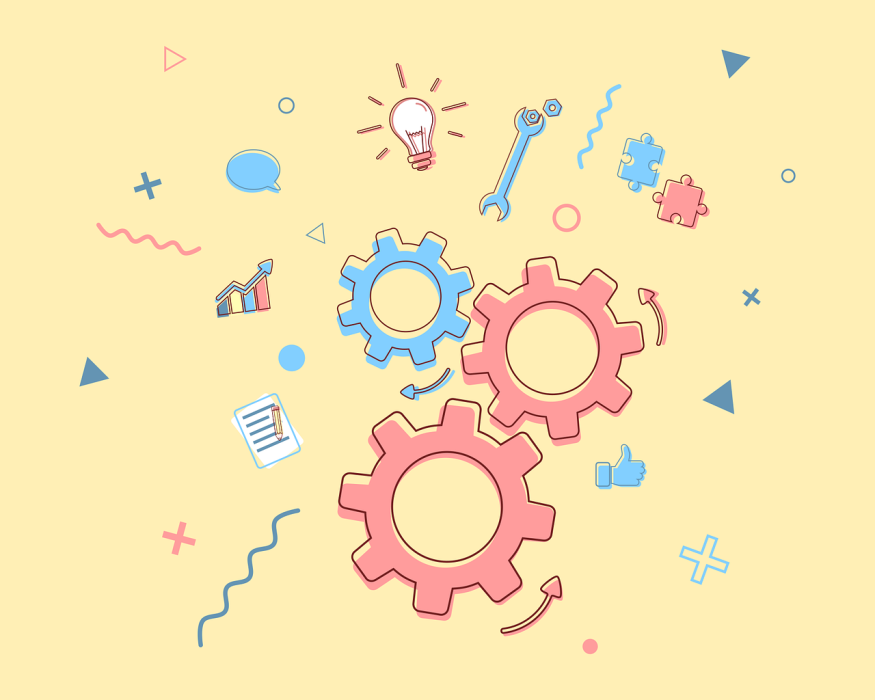
Reinforcement Learning (RL) has now become a crucial component in strategizing in the face of dynamic decision-making throughout different areas of employment. The present approach derives from the AI and ML concepts. The idea is to find the best ways to perform actions, always making sure that any behavior eventually brings the highest possible reward. The robustness and multi-functionality of RL guarantee success in developing different disciplines, such as the production of supply chains and resource management, just to list a few. This report will hence examine the different uses of machine learning, bring to light the drawbacks of implementing it, and finally examine the future perspectives of this gaining technology.
What Is Reinforcement Learning?
RL, a branch of ML, is built on an agent that can solve problems and attain decisions via actions (performance), which in turn provide feedback (outcomes) that they could learn from. These parts are controlled by agents within a world that "act" to maximize the total reward over time. Such experience is different from supervised learning as it does not involve labeled information. Rather, agents successfully learn with trial and error, trying out various tactics that they then slowly narrow down to those that are the most useful rather than following a rigid syllabus. The crucial point is, as Sutton and Barto (2018) have explained further, that RL agents do not "know what is right or wrong; rather, they learn by trying out different actions and by experiencing the repercussions."
Key Components of RL
Agent: The RL system is the agent that is the core element of. This is the part that the organization does, and it ends up learning from its encounters with the environment, which causes it to make decisions based on its observations of itself. The agent's objective is to find a strategy that will achieve an optimum sum gained over the series of actions chosen in the given situation.
Environment: The world is everything that an animal may feel, touch, perceive, and react to. Humanize: It is a solution that reacts based on the agent's actions, continuously generating new situations and challenges, which are always faced by the agent directly.
State: A state is not only a set of various parameters that determine the state but also a unique moment in time. The state has all the required information for an agent to make educated choices, unlike the other time when only one or two values can be acquired. This data can range from sensory signals of robots to market indicators of finance.
Action: Actions are the set of choices that the subject has at any given moment. Every implementation of the agent's action results in a change to the conditions of the environment. In turn, this movement is either closer or farther from the desired goal of the agent.
Reward: Reward is an important feedforward system that highlights the good extent to which the agent has competed in the given game. When making a choice, leaving a new state and as an environment displaces a reinforcement factor, cue, or information can be favorable, unfavorable, neutral, or none. The signal guides the brand-new learning course of the agent, helping it to comprehend which actions are beneficial and which are not.
Policy: The policy is a plan an agent takes advantage of to determine an action to be taken in a state. It is a linkage between states and actions through which 'Q' denotes any value for the given probability distribution. At the policy's core is a learning process that occurs in response to the agent's observation of its actions. Over time, the agent improves its strategy by reinforcement of the ones that lead to higher cumulative rewards.
Popular RL Algorithms
Q-Learning: The reinforcement-based approach represents a consistent use with continuous spaces' action.
Deep Q-Networks (DQN): Combines Q-learning with deep neural networks in the form of a Q-network that provides a powerful tool to channel complex and high-dimensional state spaces (e.g., playing video games).
Policy Gradient Methods (e.g., REINFORCE): Make the decision by directly adjusting the policy itself and cool for continuous action spaces.
Actor-Critic Methods: Classic systems which include the use of both value-based and policy-based approaches that are the basis of creation of greater stability and efficiency.
Reinforcement Learning in Supply Chain Management
In the field of production chain management, RL has shown substantial potential for the core process. Rolf et al. (2023) showcase this application's use by conducting a semi-structured literature review with a special focus on the popularity of the Q-learning algorithm and inventory management as the common application areas. The results presented, therefore, suggest the urgency of massaging priorities towards addressing large business challenges. Some scientists say that this is a challenging issue that businesses of the future must face.
Reinforcement Learning-Based Applications in Various Domains
Besides supply chain management, RL demonstrates possibilities across a broad range of areas, from healthcare to finance and entertainment. Via downloading 127 papers, Sivamayil et al. have been trying to open up the RL's exploration in energy management segments. The paper describes why RL is superior to rule-based systems, particularly in three fields of application, including renewable energy production management, smart buildings, and hybrid cars. It suggests that RL would reform these sectors completely.
Electric Power System Decision and Control
Solving sustainable issues in electric power systems, nowadays, RP applications deliver advanced solutions to the domain problems. In this paper, Glavic, Fonteneau, and Ernst (2017) shine some light on the reinforced learning method in decision-making and control within this area, however, being one of the most compelling and pathbreaking methods of algorithms for the future.
Fluid Mechanics
RL is utilized in the field of fluid mechanics. It is mostly for flow control and shape optimization, however, but not only that. In a recent survey done by Viquerat, Meliga, Larcher, and Hachem (2022), a thorough review has been provided on the state-of-the-art of RL in the mental healthcare industry. The paper compares the algorithm design made by a variety of studies and discusses the achievements made while addressing the remaining hurdles.
Building Controls
In the same context, when it comes to the performance of the building controls, the application of AI can realize impressive efficiency and sustainability. Wang and Hong (2020) revealed that applications in the real world have difficulties in fulfilling goals because they have two complicated problems: training is too complex, and control security is not reliable enough. Moreover, the survey recommends that the main focus be aimed at designing the output into practical application and boosting the efficiency of training and robustness of control.
Safe Reinforcement Learning
The idea of autonomous RL in fast-paced industrial environments, such as self-driving cars and robotics as a whole, should be at the absolute top of the list to be created, as it can be hazardous. Gu et al. (2023) survey safe RL methods, challenges, and applications. They give a systematic review with theory, method, and implementation parts. The article outlines the issues that are still unsolved in the area and gives indications of the next research directions, focusing attention on safety in RL applications.
Conclusion
Reinforcement Learning occupies the place of a sign of innovation in variable decision-making, and it manifestly exposes the range of its applications and the scope of its capabilities in many areas of life. While the examples mentioned in this section attest to the widespread functionality of the idea, they also point to the crucial issues that have to be overcome to unleash its potential. This is what the future of RL is expected to be as this technology seems to be in an endless cycle of creativity and progress, offering us a perspective of more tremendous advancements in AI and machine learning.
References
Glavic, M., Fonteneau, R., & Ernst, D. (2017). Reinforcement Learning for Electric Power System Decision and Control: Past Considerations and Perspectives. IFAC-PapersOnLine, 50(1), 6918–6927. https://doi.org/10.1016/j.ifacol.2017.08.1217
Gu, S., Yang, L., Du, Y., Chen, G., Walter, F., Wang, J., ... Knoll, A. (2023, February 20). A Review of Safe Reinforcement Learning: Methods, Theory and Applications. arXiv. https://doi.org/10.48550/arXiv.2205.10330
Rolf, B., Jackson, I., Müller, M., Lang, S., Reggelin, T., & Ivanov, D. (2023). A review of reinforcement learning algorithms and applications in supply chain management. International Journal of Production Research, 61(20), 7151–7179. https://doi.org/10.1080/00207543.2022.2140221
Sivamayil, K., Rajasekar, E., Aljafari, B., Nikolovski, S., Vairavasundaram, S., & Vairavasundaram, I. (2023). A systematic study on reinforcement learning-based applications. Energies, 16(3), 1512. Retrieved from https://www.mdpi.com/1996-1073/16/3/1512
Viquerat, J., Meliga, P., Larcher, A., & Hachem, E. (2022). A review on deep reinforcement learning for fluid mechanics: An update. Physics of Fluids, 34(11). Retrieved from https://pubs.aip.org/aip/pof/article/34/11/111301/2846714
Wang, Z., & Hong, T. (2020). Reinforcement learning for building controls: The opportunities and challenges. Applied Energy, 269, 115036. https://doi.org/10.1016/j.apenergy.2020.115036