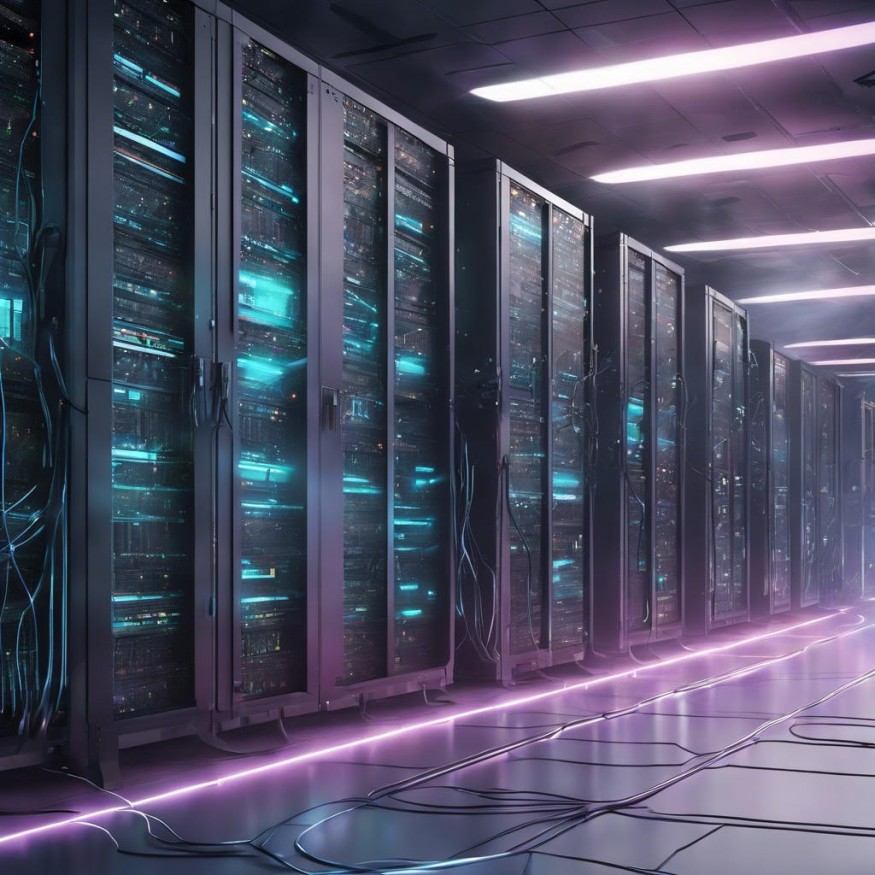
In today's world, where data reigns supreme, driving data-driven decision-making through advanced analytics and technology is crucial for success. Vinayak Pillai, a results-driven data analyst, stands at the forefront of this analytical revolution. With expertise in data modeling, system architecture, and data quality, Vinayak has significantly contributed to these fields. His journey in the tech industry is marked by relentless dedication to improving data integrity and system efficiency, positioning him as a thought leader in his domain.
From implementing innovative data quality improvements to overcoming documentation challenges, Vinayak's work has led to substantial system enhancements, improving data accuracy and operational efficiency. His detailed methodologies and hands-on approach highlight the real-world applications and benefits of robust data management strategies.
Boosting Data Quality and Architecture
Vinayak has demonstrated a meticulous approach to improving system architecture and data quality, achieving an impressive 57% enhancement. His process begins with a thorough review and understanding of the existing data model, analyzing architecture, data constraints, and relationships to identify deficiencies. This ensures that the data model accurately translates business requirements and adheres to implementation standards.
The next step involves gathering feedback and requirements from business stakeholders and leadership. Vinayak sets up meetings to understand deviations in data quality and stays updated on changes in business functions or architecture. He emphasizes, "This phase involved getting to know of any new changes in the existing business functions or architecture."
To ensure data quality, Vinayak performs in-depth data analysis, identifying anomalies and inconsistencies. He uses real-time result template mapping to measure data accuracy against ideal scenarios and defines metrics such as data absoluteness, timeliness, accuracy, continuity, and integrity. Using tools like scatterplots and Mahalanobis distance, he addresses data quality issues. He explains, "The entire missing data handling operation was performed on data-blocks which were developed by chunking the incoming data based on business-specific timestamps."
Vinayak also focuses on the scalability and adaptability of data models to future changes. He begins by understanding business needs, identifying key entities, and defining relationships aligned with business standards. His approach includes implementing normalization and denormalization, designing scalable structures, and ensuring elastic schema design using cutting-edge practices. He emphasizes, "Rolling out versions to support backward system compatibility and enabling newer features within the data-model without disrupting the original one based on the business need." Testing and scheduled maintenance are integral parts of his process to ensure that data models perform under various scenarios and remain up-to-date with security and reliability standards.
Addressing Data Flow Challenges
Encountering several challenges, Vinayak faced difficulties when documenting data flows for system improvements. He recounts, "The challenges were varied, including inferior standardization due to non-aligned data formats and nomenclatures, continuous inflow of differently structured dynamic data, and data quality issues caused by inaccuracies and missing data." To address these issues, Vinayak collaborated with leadership and stakeholders to confirm business standards and implement uniform data formats and naming structures across the entire end-to-end system. He also standardized data nomenclature and organizational standards across various inputs to manage the continuous inflow of dynamic data.
In addition to standardization issues, Vinayak faced the challenge of poor data quality. He states, "Based on the relevance of the missing data, we utilized missing-data deletion or replacement techniques like median/mode imputation and auto-encoding in deep learning." To handle continuous improvement and development scenarios, which often led to outdated documentation, he implemented automated documentation to accurately capture changes and updates.
Furthermore, the complexity of multi-integration systems was addressed by categorizing data workflows into multiple relatable modules and implementing module-wise documentation. Vinayak also emphasized the importance of adding data visualizations, providing business context, and conducting regular audits to ensure the documentation adhered to business standards and was up-to-date.

Enhancing Data Integrity
In a notable instance of improving data integrity within the automobile retailer and services industry, Vinayak significantly enhanced the effectiveness of sales and services datasets. Vinayak recounts, "The platform had a base level one common dataset which acted as the single source of truth to maintain the vehicle sales and services data during on and off acquisitions, although it was functional to a normal extent but was less productive to the growing demands of customers in different zones."
Key issues included data integrity problems due to frequent updates leading to repeated records, data redundancy with common vehicle details entered multiple times, and scalability challenges resulting in performance delays and memory overhead. Vinayak's solution involved a comprehensive data normalization process. He explains, "This step involved redesigning the entire data-model to normalize the sales and services data to separate tables," with distinct tables for sales, services, and customer information. This reorganization helped streamline data management and reduce redundancy.
Vinayak also identified relationships among datasets, enforcing data integrity constraints such as unique constraints and foreign-key constraints. Essential measures were precomputed and denormalized for better performance and report generation. Regular audits and versioning were conducted to manage acquisition data and process changes effectively, resulting in enhanced performance, increased scalability, hassle-free maintenance, and improved data integrity and security.
To measure the effectiveness of system improvements and data quality enhancements, Vinayak employed methodologies such as defining Service Level Agreements (SLAs) and measuring KPI alignment with business goals. He elaborates, "This process involves setting up benchmarks to compare the performance of the system and track areas having a scope for improvement based on business objectives." He conducted thorough quality assurance testing and impact analysis and facilitated continuous improvement through surveys, retrospectives, and beta-launch reviews to gather ongoing user feedback and make necessary adjustments.
Spotting Architectural Weaknesses
Recalling a specific scenario, Vinayak identified critical areas for architectural improvements during a system migration for a retail client. The homegrown system, reliant on an on-prem data repository, struggled to handle a surge in orders during festive times and required integration with the acquired company's Order Management System (OMS).
To address these issues, Vinayak began by analyzing the existing system and pinpointing key areas for improvement. He discovered several issues, including scalability problems, poor user experience, integration complexity, lack of disaster recovery mechanisms, downgraded performance during peak intervals, and insufficient data security. He implemented data partitioning and caching to distribute the load across multiple servers, enhancing data retrieval performance.
Additionally, Vinayak designed and deployed a high-availability architecture with load balancing to ensure uninterrupted operations and data accuracy. He transitioned from a traditional on-prem architecture to a microservices architecture to improve scalability and fault isolation. "This resulted in a successful and improved architectural implementation for the retail client," Vinayak concludes, highlighting the positive impact of these comprehensive architectural improvements.
Transitioning System Architecture
Playing a crucial role, Vinayak transitioned a major retail client from an inefficient AS-IS system to an optimized TO-BE system. The project involved migrating data from a local retail company to an acquired gaming company's system. The original setup, relying on an on-prem environment, suffered from data integrity issues, poor scalability, and performance bottlenecks during peak times. Vinayak recounts, "The homegrown system of the acquired company lacked scalability, failing to perform during an upsurge of increased number of requests."
To address these challenges, Vinayak conducted a thorough analysis of the existing system, identifying areas needing improvement. He defined the TO-BE state based on business requirements, data volume, and technological needs. This included designing a comprehensive data-flow model and optimized algorithms. Vinayak states, "We identified the gaps between the AS-IS system and the TO-BE system and laid out migration strategies." He set up data pipelines, ensured system integrations, and conducted extensive quality assurance testing. The successful implementation of these steps resulted in a smooth transition to a more scalable, user-friendly, and secure system architecture. Regular feedback sessions and continuous improvement loops were established to maintain the system's effectiveness and adapt to future changes.
Collaborating for Success
Employing a comprehensive approach, Vinayak collaborates with teams and stakeholders to ensure system improvements align with overall business objectives. He initiates the process by setting up stakeholder discussion sessions to understand the requirements from a business functional perspective. This step is crucial for capturing the diverse viewpoints of different teams. By rotating requirements across various teams, he ensures that all functional perspectives are considered, fostering a holistic approach to system improvements.
To maintain alignment with business objectives, Vinayak sets up continuous improvement meetings to solidify requirements and ensure everything falls into place from both business and functional standpoints. He emphasizes the importance of transparency and feedback throughout the process, stating, "Releasing prototypes and demos to the stakeholders ensures that the requirements and the delivery are up-to-code." Regular feedback sessions are conducted to guarantee end-user and stakeholder satisfaction. Furthermore, Vinayak ensures thorough documentation to provide transparency from a design and build perspective and sets up post-deployment reviews with leadership to gauge the effectiveness of the system builds and identify areas for improvement. This structured, inclusive approach ensures that system improvements are effectively aligned with business goals.
For professionals looking to make a similar impact in the field of data and systems, Vinayak's journey offers valuable lessons in resilience, thoroughness, and a commitment to excellence. His work exemplifies the importance of having a strong foundational understanding of both technical and business needs and the ability to translate those needs into reliable, scalable solutions. By following his example, other professionals can strive to achieve similar levels of success and innovation in their own careers.