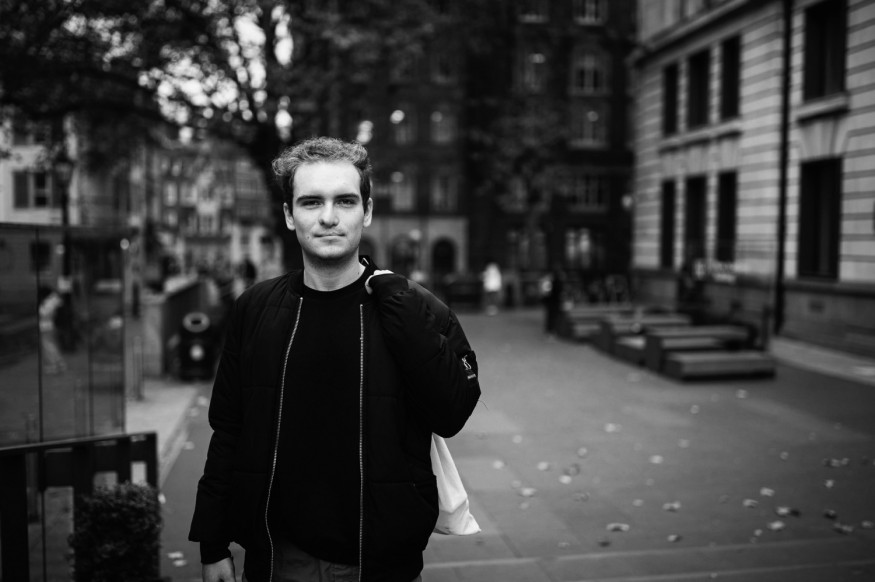
It's no surprise that large language models (LLMs) are set to revolutionise how we work. With the recent release of OpenAI's ChatGPT API, businesses now have the opportunity to integrate this powerful tool into their workflows, unlocking numerous benefits and automating significant portions of their operations. However, as Boris Surov, who combines tech and business leadership expertise, points out, it is crucial to identify tasks best suited for LLMs and understand the human role in their integration. In this article, together with Boris, we explored the strategic benefits of incorporating LLMs into business workflows, identified tasks that are prime candidates for these models, and emphasised the importance of human oversight in monitoring AI-generated results.
Maximising Human Capital Efficiency
According to Surov, "the most valuable resource of any organisation is its people." However, routine tasks that are essential to business operations often force teams into repetitive activities. Integrating LLMs can automate many of these tasks, significantly reducing the need for constant human intervention. This allows teams to focus more on meaningful, challenging work. "Start by identifying routine tasks where physical labour isn't required," suggests Surov, "and consider how they can be 'delegated' to an LLM."
Real-Time Data Analysis
Language models excel at processing and analysing large volumes of diverse data in real time. Businesses can input various types of data or large documents into an LLM—ensuring that personal data is excluded—and generate nearly any type of analysis or evaluation. This capability enables companies to respond to changes quickly, make informed decisions, and gain valuable insights from multiple data sources. Surov explains that LLMs are particularly useful for analysing customer feedback, market trends, and operational metrics, thus enabling faster and more informed decision-making.
Automating Internal Reporting and Optimising Communication
Language models can also streamline communication processes within a company. For instance, integrating OpenAI's API with an internal analytics tool allows for the effortless generation of reports or presentations with a well-crafted prompt. Additionally, by uploading help resources or internal documentation, businesses can create dynamic chat systems for both customers and employees, providing instant answers to dataset-based questions. "This not only streamlines communication but also enhances the overall efficiency and responsiveness of your support systems," Surov notes.
Effective Task Delegation Between AI and Humans
For optimal efficiency, it is essential to delegate tasks between AI and human workers properly. Surov emphasises the importance of distinguishing tasks that are better suited for AI from those requiring human intervention. "LLMs are algorithms capable of analysing and generating text in natural language," Surov explains. "Any task involving data analysis to generate responses is a good candidate for a language model." Routine, monotonous operations requiring high accuracy and speed—such as data processing or process automation—are well-suited for LLMs. However, tasks involving creativity, uncertainty, or complex decision-making are better handled by humans.
Practical Applications of Language Models
LLMs can handle a wide range of text-based tasks across various company functions. Surov provides several practical examples:
- Marketing, Advertising, and Content Creation: LLMs can generate content such as advertising texts, product descriptions, and social media posts. By providing detailed instructions on content format, style, and examples, businesses can obtain high-quality texts ready for editing and use.
- Customer Support: With predefined guidelines, LLMs can automate a significant portion of the quality control process in customer support. Businesses can upload support guidelines, product documentation, and evaluation criteria into the model, automating the analysis of team performance.
- Internal Operational Processes: For tasks like filling out text documents or product information, LLMs can reduce the need for human resources. Reporting, operational metrics analysis, and other quantifiable performance data can be automated, allowing businesses to generate weekly summaries or in-depth reports with ease.
Data Collection and Human Oversight
Successful integration of LLMs requires careful data collection and human oversight. "Focus on collecting and organizing the necessary data—scripts, tables, and examples for model training," advises Surov. The complexity of the task will determine the level of data and training required. For instance, automating social media content may require simple prompts, while evaluating customer sentiment may necessitate more sophisticated API integration and data anonymisation.
Testing the model's output is critical. "Expect low-quality results initially, as it typically takes several iterations of improving instructions and input data format to achieve optimal results," Surov warns. Even with well-optimised prompts, LLMs can still make mistakes, underscoring the importance of organising a process for checking and editing AI-generated results. Human oversight is necessary for all tasks but becomes especially critical for complex, high-risk operations.
Conclusion
As was revealed by Boris Surov, language models can significantly enhance efficiency by automating routine tasks and improving data processing—if approached correctly. The key to successful integration lies in thoughtful task delegation between AI and humans, with clear criteria for evaluating their work. As Surov emphasises, LLMs are designed to complement, not replace, human labour, particularly tasks requiring creativity, interpersonal interactions, and high-risk decision-making should be noted by other industry leaders with an ambition to keep up with the ever-changing tech market. Human oversight is essential, ensuring that AI-generated results are accurate and reliable, especially for complex tasks where the cost of errors is high.