Brain-machine Interface (BMI) monitos and evaluates brain signals, transforms them into commands, and transfera these commands to an external device, which subsequently performs the required action. A mind-controlled wheelchair is one example of an output device that might help paralyzed people become more mobile, as per PubMed Central.
The patients had quadriplegia, also known as tetraplegia, a kind of immobility that involves all four limbs and is caused by a cervical spine injury. The introduction of brain-controlled wheelchairs in previous seasons has been a huge development, but they still need invasive brain-computer implants. Other ways rely on clunky workarounds, such as looking at a light sensor in the location one wishes to travel.
Despite advancements in BMI research, its value in a clinical or "real-world" situation remains unproven at this time. How simple is it to operate? Is there a degree of expertise required such that the person utilizing BMI is training to control the device while the BMI is gaining from the individual's brain?
Brain-Controlled Electric Wheelchair
A recent study by the University of Texas in Austin academics led by Professor José del R. Millán inquired about the importance of BMI skill learning in managing a non-invasive BMI-operated electric wheelchair within real-world contexts. According to Millán, they show that reciprocal learning of both the user as well as the brain-machine interface algorithms is crucial for users to properly use such wheelchairs.
Millán alongside colleagues included three tetraplegic individuals in their research, who received training three times each week for up to five months. Electroencephalography (EEG) data were acquired from every individual employing a skullcap, which has been subsequently transformed into wheelchair operating commands. The patients were instructed to consider manipulating their parts of the body to control the movement of the wheelchair, such as turning left with both hands and right with both feet.
The participants displayed comparable levels of accuracy (43-50%) while utilizing the BMI-controlled wheelchair at the start. However, they discovered that this accuracy may be enhanced during the training session. By the completion of the training, participant one's accuracy had climbed to 95%, while participant three's accuracy had reached 98% midway through the program.
As stated by the researchers in the press release, the gains in accuracy correspond with advances in component discriminant, which refers to the algorithm's capacity to discern between brain signals that indicate a left turn even against a right turn. The data indicate that better feature discrimination is also a component of the participants' learning; the EEG results indicated brainwave pattern modifications as accuracy improved. Using the EEG data, the patient has consolidated the ability to modulate distinct portions of their own brains to establish a sequence for 'go left' or a distinct configuration for 'go right,' adds Millán. Scientists hypothesize that the learning process of the participants resulted in brain remodeling.
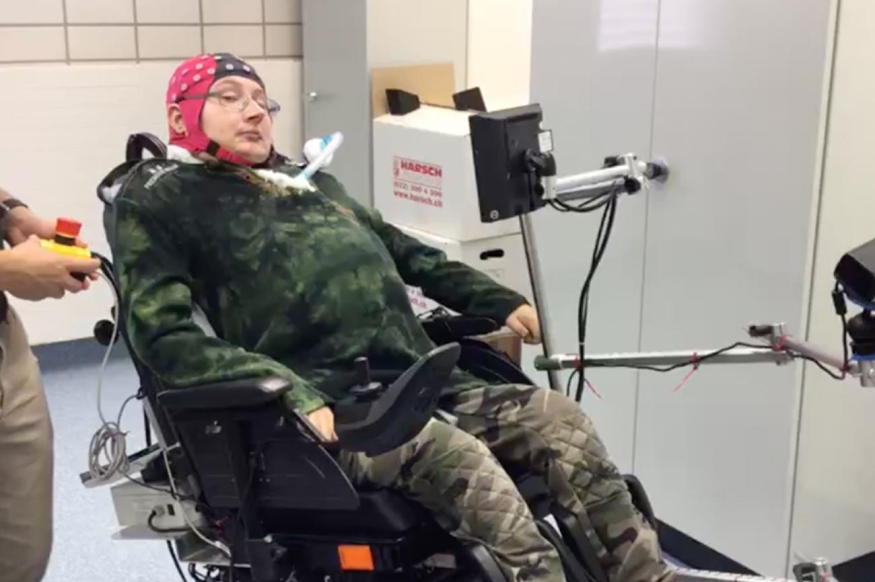
ALSO READ : Tech Companies Now Offering Mind-Reading Devices; Are Businesses, Corporations Ready For This Innovation?
Simulation of the Brain-Controlled Wheelchair
Throughout the training, participant two had no notable changes in their brain activity patterns. Instead, within the first few sessions, their accuracy rose somewhat before maintaining consistency throughout the training time. This, according to Millán, shows that machine learning itself is unable to properly manage mind-controlled equipment in all patients.
All selected participants were expected to drive the wheelchairs through a congested room, dodging obstacles such as a space divider and hospital beds, towards the end of training. Subjects one and three successfully finished the task; however, participant two failed.
As said by Millán, it appears that strong brain-machine interface management that allows someone to conduct reasonably complicated daily activities like operating a wheelchair in a native surrounding involves some neuroplastic rearrangement in our cortex. Consistency may be essential when using a mind-controlled wheelchair. Although participant one's performance towards the conclusion of training was outstanding, the researchers claim that they first struggled with the gadget.
Despite the study's modest sample size, this is one of the first to investigate the possible adaptation of non-invasive BMI technologies in tetraplegic patients. Millán and collaborators are currently concentrating on brain signal data to investigate why subject two didn't experience the learning outcomes and what treatments could be effective in the future to aid patients suffering from the learning process. Their collaborative study was published in the online academic journal iScience.
RELATED ARTICLE : Brain-Computer Interface That Can Be Controlled Hands-Free May Benefit People With Paralysis
Check out more news and information on Technology in Science Times.