Dr. F. Perry Wilson from Yale School of Medicine discusses the cutting edge of modern medicine, which uses an artificial intelligence model to guide care. He explains how AI in medicine has a major Cassandra complex.
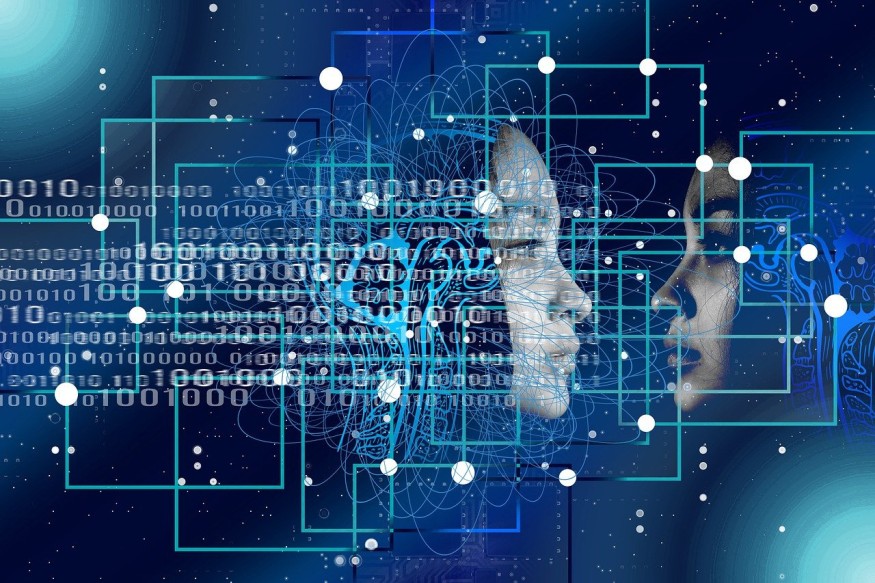
What is Cassandra Complex?
In Greek mythology, she was the daughter of Priam, the king who ruled Troy when the Greeks attacked it. Due to her beauty, Cassandra attracted the attention of god Apollo, the son of Zeus. He bestowed her the gift of prophecy as a love gift, but she refused his attention, making him angry. As a result, Apollo cursed Cassandra that she would always prophesy the truth, but nobody would believe her.
When her brother Paris set off to Sparta to abduct Helen, Cassandra warned him about his actions' effects on their city's downfall. Troy was known for its towering walls, which made it seem unassailable, but that did not stop the Achaeans from making landfall. Cassandra foretold the city's destruction, and the famed fall of Troy was even mentioned in the Iliad and the Aeneid.
The term 'Cassandra complex' was first used in 1949 when French philosopher Gaston Bachelard identified a modern psychological syndrome where urgent, fact-based alarms are dismissed and ignored. This complex has a wide-ranging context, from psychology science, and philosophy.
READ ALSO : Can ChatGPT Be Better Than Human Doctors? AI Advancements Show Potential in Redefining Medicine
Cassandra's Problem in AI
Electronic health records allow the collection of volumes of data orders of magnitude greater than what has been gathered before. Different algorithms can crunch all that data to make predictions about anything. It can be used in deciding whether to transfer a patient to the intensive care unit, whether gastrointestinal bleeding will need an intervention, or whether a patient will likely die the following year.
Studies in this area depend on retrospective datasets. As time passes, better algorithms and more data have led to better predictions. In some cases, machine learning models have even achieved near-perfect accuracy, or Cassandra-level accuracy, like in the reading of chest X-rays for pneumonia.
However, as the story of Cassandra teaches us, even perfect predictions seem useless if no one believes in them or if people do not change their behavior. This is the central problem of AI in medicine today. Many people focus on the accuracy of the prediction but fail to remember that high accuracy is just table stakes for an AI model to be useful. It needs to be accurate, and its application has to change patient outcomes.
The best way to determine the role of an AI model in helping patients is to treat it like how a new medication is handled. It must also be evaluated through a randomized trial. This is what the researchers did in a study by Shannon Walker of Vanderbilt. They investigated how an automated prognostic model embedded in the electronic medical record can help in preventing hospital-acquired venous thromboembolism (HA-VTE) among hospitalized children and adolescents. It was found that despite the use of an accurate and validated predictive model for HA-VTE, substantial reluctance was shown by the primary clinical teams in initiating thromboprophylaxis as recommended.
RELATED ARTICLE : AI Doctor? ChatGPT Nearly Passes US Medical Licensing Exam
Check out more news and information on Artificial Intelligence in Science Times.