Scientists at the University of Cambridge, in conjunction with Jaguar Land Rover (JLR), have devised an adaptive algorithm aimed at enhancing road safety by anticipating optimal moments for drivers to interact with in-vehicle systems or receive messages like traffic alerts.
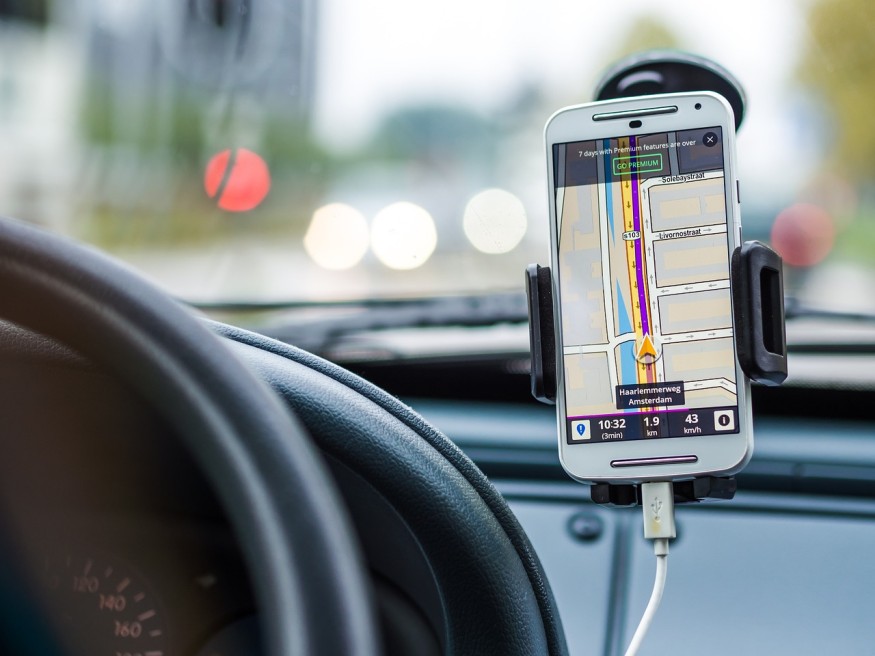
A Road Safety Machine Learning Algorithm for Drivers
Scientists reported in the paper, titled "Driver Profiling and Bayesian Workload Estimation Using Naturalistic Peripheral Detection Study Data" published in the journal IEEE Transactions on Intelligent Vehicles, that the machine learning algorithm utilizes a mix of on-road experiments and machine learning, alongside Bayesian filtering techniques, to consistently gauge driver "workload."
The algorithm exhibits dynamic adaptability, responding in real-time to changes in conditions and driver behavior during tasks like navigating unfamiliar areas or daily commuting, providing a promising tool for enhancing road safety. This adaptability ensures swift responses to variations in the driver's behavior, status, road conditions, type of road, or driver characteristics.
This data can then be integrated into in-vehicle systems, including infotainment, navigation, displays, and advanced driver assistance systems (ADAS). The algorithm allows for customized driver-vehicle interactions, prioritizing safety and refining the overall user experience.
For instance, alerts are strategically timed during periods of low workload, ensuring that drivers can maintain full concentration during more demanding driving situations.
Dr. Bashar Ahmad, co-first author from Cambridge's Department of Engineering, highlighted the increasing availability of data to drivers but emphasized that it poses a significant risk to road safety due to rising driver demand. He emphasized the importance of knowing the driver's status to ensure that the vehicle provides information safely and practically.
READ ALSO: Elon Musk Anticipates Tesla's Imminent Achievement of Full Self-Driving Capability 'Later This Year'
Calculating Driver Workload
Driver workload, a dynamic aspect, varies in challenging situations such as driving in unfamiliar areas or heavy traffic. The challenge for car manufacturers is to assess driver occupation accurately and initiate interactions or messages when the driver is receptive.
While existing algorithms use eye gaze trackers and biometric data, the Cambridge researchers sought a method utilizing standard driving performance signals, like steering and braking data, available in any car.
The team designed a modified Peripheral Detection Task, allowing drivers to indicate low workload during an experiment, where video analysis and machine learning identified high workload scenarios.
The adaptive algorithm developed adapts on the go using Bayesian filtering techniques, accommodating different road types, conditions, or drivers using the same car.
By incorporating this algorithm, vehicles can provide notifications during low-workload scenarios, minimizing distractions in demanding situations. This technological advancement represents a significant step in enhancing road safety by ensuring drivers stay focused, particularly in challenging driving conditions.
In today's era of increased in-vehicle connectivity, monitoring and adapting to driver workload are crucial for road safety. This innovative technology prioritizes safety, optimizes driver-vehicle interactions, and has the potential to greatly improve the overall driving experience while minimizing distractions on the road.
RELATED ARTICLE: 'Uber Bot' Replacing Human Drivers; Six-Wheeled Delivery Robots Rolling Out in Dadeland, Miami
Check out more news and information on Artificial Intelligence in Science Times.