Exploring Mars has always been one of the fascinating subjects for scientists and space enthusiasts. As experts continue to learn more about this planet, the possibility of human colonization of Martian terrains becomes more realistic. However, one of the key challenges in this goal is finding suitable habitats for future astronauts to live in.
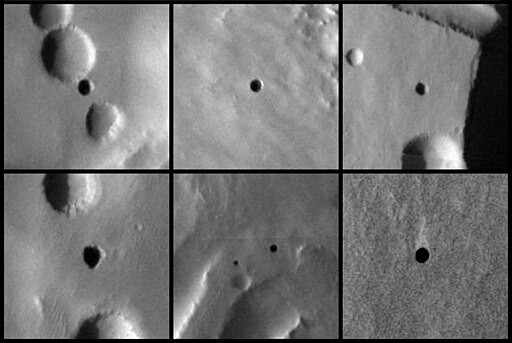
Challenges in Detecting Cave Entrances
Compared to Earth, the Martian surface is hostile and unforgiving, yet scientists believe it could be habitable. Some of the most interesting places to explore on Mars are cave entrances formed from collapsed lava tubes. These areas offer shelter for future human explorers and can be a great place to search for biosignatures of microbial life.
The caves in the red planet are created by lava tubes formed by flowing lava on ancient Mars. As the outside of the flowing lava cooled and solidified into a ceiling and walls, the interior portion stayed molten and kept flowing. The lava eventually flowed out of the tube in a downslope direction and left the tube intact and open. However, cave entrances are difficult to locate, especially from orbit, since they blend in with the dusty surroundings.
In the past observations of the red planet, most detections of potential cave entrances (PCEs) have come from a manual review of visible satellite imagery, with images captured by the Mars Reconnaissance Orbiter's (MRO) Context Camera (CTX) and High-Resolution Imaging Science Experiment (HiRISE) cameras. From that manual review, a Mars Global Candidate Cave Catalogue (MGC3) database contains the coordinates and brief descriptions of over 1,000 identified PCEs on Mars.
On a planet-wide scale, manual review of satellite imagery for Martian cave detection is far from efficient. This is due to the time constraints associated with reviewing a large dataset.
CaveFinder CNN Model
To address this challenge, scientists developed a new machine-learning algorithm to quickly scan Martian surface images to search for potential cave entrances. Durham University researchers Thomas Watson and James Baldini made it possible using a convolutional neural network (CNN) called CaveFinder.
The new CNN was trained to identify PCEs from captured images of the Martian surface. It uses images from the MGC3 catalog taken from the Tharsis and Elysium regions on Mars, the areas with the highest concentrations of volcanoes. It also achieved a test accuracy of 77% after the training period.
Using images in four different regions on Mars, it identified 61 new entrances. It found the PCEs highlighted by Watson and Baldinifor have specific qualities that make them promising for further research. Machine learning solves the problem in manual review of satellite imagery by reducing the dataset to include only imagery computationally determined to contain a PCE.
In the future, the researchers plan to increase the size of their training dataset. Some of the plans to improve the accuracy of CaveFinder is the use of thermal imagery alongside visible data and higher resolution images from a future Mars orbiter.
RELATED ARTICLE : Radar Survey Reveals Giant Layers of Water Ice Buried in Mars' Medusae Fossae Formation
Check out more news and information on Mars in Science Times.