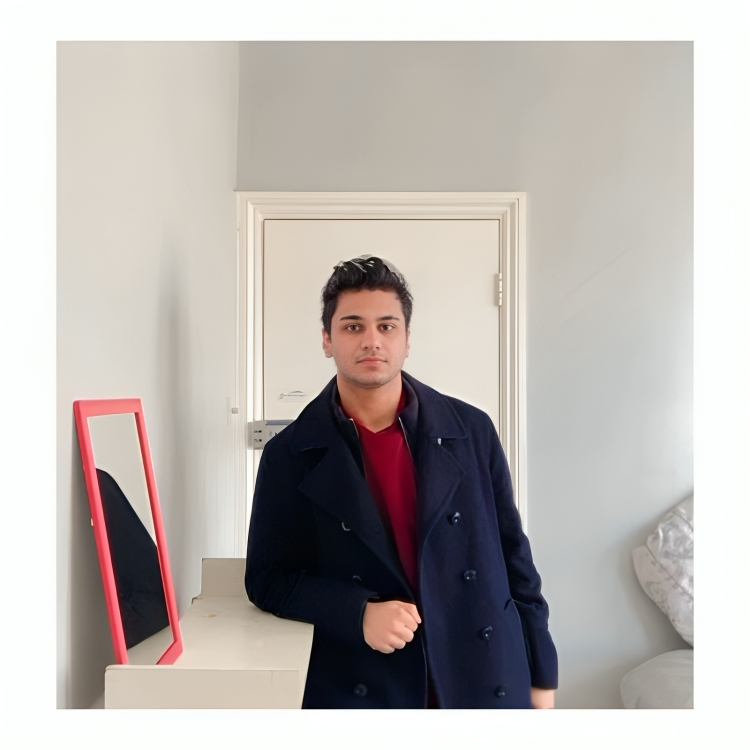
GPT Agents, short for Generative Pre-trained Transformer Agents, bring a paradigm shift in artificial intelligence (AI), particularly within the domain of Natural Language Processing (NLP). These agents, use transformative architecture of generative pre-trained transformers, a huge advancement in automated task execution.
GPT Agents use Transformer architecture, which is meticulously designed for processing sequential data. This architectural framework allows these agents to dissect and scrutinize core task objectives—executing tasks methodically through iterative processes. Analyze core objectives and complete tasks step by step. It also has the power to access the internet, memory, and payment forms.
Transformers are advanced models in Natural Language Processing (NLP) and are seen as an upgrade from the old encoder-decoder setup. Unlike the encoder-decoder architecture, which heavily depends on Recurrent Neural Networks (RNNs) to process sequential data, Transformers take a different approach.
Instead of using recurrency like RNNs, Transformers focuses on understanding context and meaning by examining how different parts relate to each other. They primarily rely on a mathematical method known as attention to achieve this task.
How do GPT Agents work?
The process begins with the user assigning a task to the GPT agent. Subsequently, the task is queued for execution and passed through the Execution Agent before being stored in the agent's memory. Contextual information is integrated with the task objective, drawing insights from the knowledge base. The Task Creation Agent then generates additional tasks based on both the objective and contextual understanding. These tasks are received by the Task Queue, where the Task Prioritization Agent arranges them in order of priority. This cyclical process continues iteratively until the user's objective is fulfilled.
Applications of GPT Agents:
1. Personal Assistance and Web Access:
- Conducting web searches, managing finances, and scheduling tasks.
- Facilitating travel bookings and health monitoring.
2. Customer Support:
- Handling end-to-end inquiries through chatbots.
- Offering support across websites, applications, and messaging platforms. Cross-collaboration to complete the inquiry.
3. Financial Management:
- Conducting end-to-end. Credit card assessments and ensuring compliance.
Why GPT Agents Don't Work (Yet!)
Something that I recently learned from Dharmesh Shah's newsletter—error rates endemic to language models pose a challenge, particularly when compounded across multiple task invocations. A rigorous probabilistic analysis explain how these error rates on aggregate task success. A conservative 90% success rate per invocation, the cumulative success probability for a succession of 10 invocations diminishes exponentially.
The probability of overall task success is just computed as the product of individual success probabilities, in this case it would be mere 35% likelihood of task completion.
How to Fix the Problems
Although in a general sense, Agent AIs currently face limitations, it's feasible to develop specialized agents tailored for specific tasks. This targeted approach contrasts with creating all-encompassing agents like AutoGPT. By focusing on specific domains, developers can significantly reduce error rates.
Here are some strategies to enhance the effectiveness of these custom agents:
- Begin with a precise, use-case-specific system prompt to initiate tasks. Understanding the agent's supported goals improves the likelihood of selecting the right tasks initially. Defining tasks upfront, if feasible, can also be beneficial.
- Incorporate human assistance when needed. Recognizing tasks better suited for human intervention allows the agent to seek human input for challenging questions beyond its capabilities.
- Integrate mechanisms for human feedback. Instead of running tasks autonomously, periodically soliciting human feedback ensures alignment with the correct course. Advanced agents utilize Reinforcement Learning from Human Feedback (RLHF) to improve performance over time based on user input.
In conclusion, GPT Agents signify a groundbreaking advancement in AI, particularly in the realm of Natural Language Processing (NLP), through their innovative use of generative pre-trained transformer architectures. These agents exhibit a transformative capability to methodically execute tasks by analyzing core objectives and leveraging contextual understanding. With access to vast knowledge bases and the ability to process sequential data through attention mechanisms, they promise a wide array of applications ranging from personal assistance to customer support and financial management.
Connect with Ronak Joshi on Linkedin — https://www.linkedin.com/in/ronak-joshi-903b95298/
About
Ronak Joshi has successfully built and scaled more than six businesses without any external funding. Collectively, my products ( 30+) boast over 500,000 users.
Hireinfluencer, ReferEasy, Consultme, Thesubscription, and more.