Millions of people die every year because of the spread of bacteria that are not killed by antibiotics. Researchers are using AI to find new medicines, which could completely change the field.
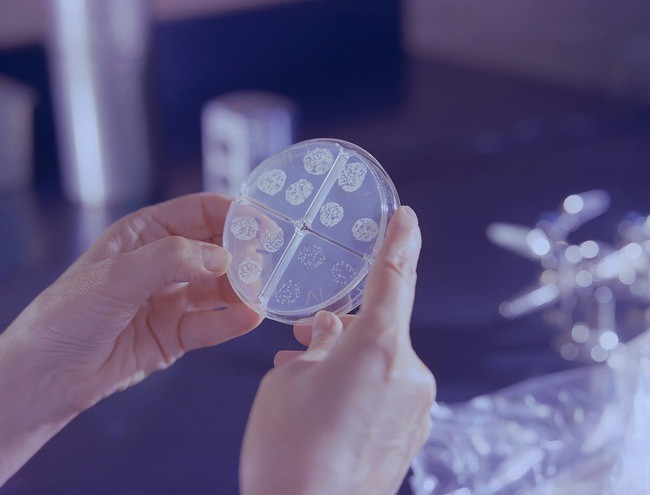
Use of AI to Discover New Antibiotics
Cesar de la Fuente from the University of Pennsylvania and his team of people from different fields have made an AI tool to fight this threat. Published in the journal Nature Biomedical Engineering, their study shows how to use deep learning methods to recognize and examine over 10 million molecules from extinct and living species. This method aims to find new antibiotic substitutes that might completely change the industry.
The old ways of making new preclinical drug prospects take a long time and cost much money. It usually takes about six years.
De la Fuente's deep learning method cuts this time and cost by a huge amount, finding thousands of possibilities in just a few hours. Many of these options have shown promise in animal models before being tested on humans.
Since starting at Penn in 2019, de la Fuente has been investigating whether machines can speed up the process of finding new antibiotics by collecting biological data. He says biology is just a source of data that AI can use to find useful new chemicals. The team first used simple methods to look through individual proteins for small antibiotic molecules within their amino acid sequences.
As computers got faster, they could go from mining single proteins to mining whole proteomes. Scientists used this method to find thousands of new antibacterial molecules in the proteomes of humans, Neanderthals, and Denisovans, who lived a long time ago. They also set the goal of mining for all known extinct creatures, leading to the idea of "molecular de-extinction."
A New Era in the Search for Antibiotics
The team discussed a deep learning model called Antibiotic Peptide de-Extinction (APEX). APEX can take samples of hundreds of proteomes from different species throughout evolution.
This helps find the best antibiotic candidates from wooly mammoths and ancient sea cows. Marcelo Der Torossian Torres, a postdoctoral researcher in the de la Fuente Lab and co-first author of the work, stressed the importance of training APEX with a standard dataset to ensure the model was accurate and useful.
The APEX system uses attention networks and recurrent neural networks to find antimicrobial peptides within proteins. Recurrent neural networks do well with sequences; attention networks concentrate on particular regions of the protein structure that are probably involved in antibacterial action.
APEX outperformed the benchmark models. The system examined 10,311,899 peptides and identified 37,176 sequences predicted to have a wide range of antibacterial activity, including 11,035 sequences not present in organisms.
The success of several of these sequences in preclinical animal models advanced their prospects for clinical studies and medical uses. Most ancient peptides, interestingly, depolarized bacterial cell membranes in a novel way. This novel approach raises the possibility of an alternative strategy for viral illness treatment.
Over the past five years, the computer work done in de la Fuente's lab has made it much faster to find new drugs. What used to take years to do the old-fashioned way can now be done in hours with AI.
Molecular de-extinction seeks to revive molecules to address contemporary biological and medical challenges, including antibiotic resistance. This new approach may result in a future in which AI is essential to combating drug-resistant bacteria and developing new antibiotics.
RELATED ARTICLE : Novel AI Can Predict COVID-19 Vaccine Attitude Using Integrated System of Machine Learning, Math of Human Judgment
Check out more news and information on Artificial Intelligence in Science Times.