Scientists at the RIKEN Center for Computational Science recently used a "butterfly attractor" computer system to show that extreme weather phenomena can be regulated and modified by making minor adjustments to variables in the weather system.
A report from the European Geosciences Union specified that decades of global research had ignited a big question ---if the weather can indeed be controlled.
This new study stated this might soon be the world's new reality. The developers did this using the said system in chaos theory, which has one of the two states, similar to a butterfly's wings, and shifts back and forth between the two states depending on tiny changes in some conditions.
The study's findings have promised multiple applications in the future in which weather events can be regulated, including impacts of climate change.
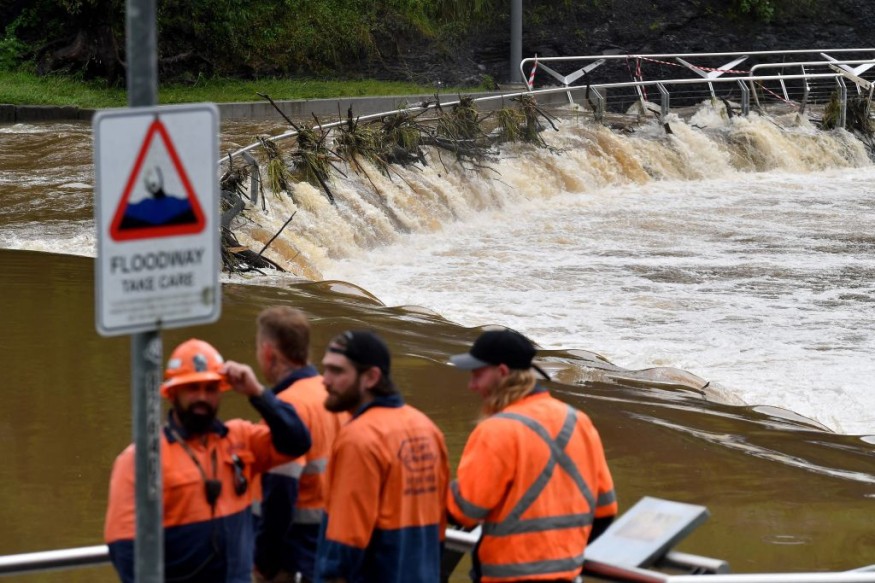
The Butterfly Attractor
The butterfly attractor was initially proposed by Edward Lorenz, a mathematician, and meteorologist, one of the founders of modern chaos theory.
Describing the new approach, Lorenz said even the most minute, butterfly-scale changes to this computer weather model "caused a range of weather outcomes from bright skies to raging storms," without predicting the final outcome.
Since the meteorologist first presented his work in the 1970s, his theory about the butterfly effect became very popular and stays so, even at present. It comprises the metaphor that a butterfly flapping its wings in Brazil can bring a tornado to Texas.
Modern Chaos Theory
The RIKEN researchers started investigating the chaos theory of Lorenz to develop realistic possibilities for mitigating weather occurrences such as torrential terrain, the study published in the journal, Nonlinear Processes in Geographics specified.
They ran one weather simulation to function as "nature" itself or the control side. Then, they ran other simulations using small variations in variables in explaining the convection, how heat is moving through the system.
As a result, the scientists found that they could control nature in a chosen regime minus shifting to the other, for instance, in a selected wing of Lonrenz's butterfly attractor, by adding small changes to nature.
According to the RIKEN Center for Computational Science's Takemasa Miyoshi, who led the research team, they have successfully built a new theory and methodology to investigate the controllability of weather.
Based on observation of the system simulation systems used in past research, the researchers were able to design an experiment to examine predictability, on the assumption that the actual values or nature cannot be changed, but instead, but rather that "we can change the notion of what can be changed."
Looking Into the Controllability of Weather
Even though weather forecasts have reached high accuracy levels because of supercomputer-based simulations and data assimilations, researchers have long hoped to be able to control the weather.
The RIKEN report specified that climate change has "further intensified researchers in this area," due to the increased risk of extreme weather occurrences such as storms and torrential rains.
According to Takemasa, this research opens the path to research into the controllability of weather and, soon, could lead to weather control technology.
He explained, if realized, this study could help in the prevention and mitigation of extreme windstorms like torrential rains and typhoons, whose risks are increasing with climate change.
Related information about the butterfly attractor is shown on Josh Kastorf's YouTube video below:
RELATED ARTICLE : Humans Cause Climate Change: NASA Show Direct Evidence from Calculation
Check out more news and information on Weather in Science Times