While looking for life beyond Earth, researchers have few options to gather samples on Mars or elsewhere or to use remote sensing tools. The multidisciplinary research, titled "Orbit-to-ground framework to decode and predict biosignature patterns in terrestrial analogs" published in the journal Nature Astronomy, explored the scant life hidden away in salt domes, boulders, and crystals at Salar de Pajonales at the border of Chile's Atacama Desert and Altiplano.
SETI Institute Senior Research scientist Kim Warren-Rhodes and colleagues then trained an AI model to recognize patterns and rules linked to their attributes to detect biosignatures up to 87.5% accurately and decrease the search area by up to 97%.
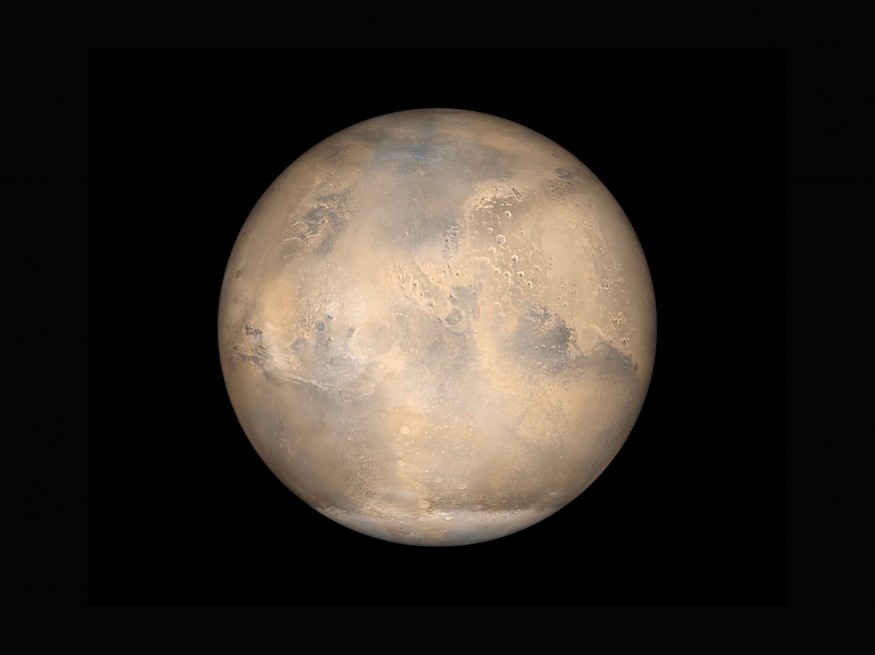
New Way To Optimize Search For Signs of Life
Rhodes said that their framework allows them to combine statistical ecology with machine learning to find and predict patterns in which nature survives and distributes itself on Earth. They hope that other astrobiology teams adapt their approach to mapping habitable environments and biosignatures on other planets.
Vice reported that the new deep learning framework was trained to find biosignatures in an environment with extremely cold temperatures to at least mimic conditions on Mars. The AI tool was able to increase the likelihood of detecting signs of life in such extreme environments to make it almost nine times as effective as random searches.
Space agencies have sent orbiters and rovers to the Red Planet's locations which they suspect were sculpted by water, a key ingredient for life as humans know it. Although it has given a rough idea of where to look for biosignatures, narrowing down the search area is a more challenging task.
To address this, Warren Rhodes and colleagues used AI to predict biosignature patterns. They noted that there is an abundance of data from these orbiters and rovers to characterize global and regional habitability, but lesser information about microbial habitats and biosignatures.
Through their new paper, researchers aimed to fill the gap by training a deep learning system to predict the presence of biosignatures at Chile's Salar de Pajonales.
Even though it is not perfectly the same as Mars, it exhibits features from both physical and biological processes that are crucial in finding signs of life on Mars, like a fractal-like ridge network, patterned ground, and shrinkage crack terrains of abiotic origin.
Testing the AI Under Different Extreme Environments on Earth
EurekAlert! reports that the team also plans to test the AI's ability to predict the location and distribution of stromatolite fossils and halite microbiome to see if similar rules apply to other yet similar natural systems.
These entirely new ecosystems include hot springs, permafrost soils, and rocks in the Dry Valleys. As they gather more data, hypotheses about the beginning of life in extreme environments will be tested, as well as biosignature probability blueprints for key analog ecosystems and biomes on Earth will be inventoried.
Nathalie A. Cabrol, the PI of the SETI Institute NAI team, noted that successfully integrating datasets at vastly different resolutions from orbit to the ground is also an important aspect of their study aside from the high-rate detection of biosignatures. Their study demonstrated a pathway for utilizing AI in assisting scientists in the search for biosignatures in the universe.
RELATED ARTICLE: Search of Life on Mars Continues: Fossil Mimics, False Positives Makes Research Harder
Check out more news and information on Space in Science Times.